AI Analytics Tools for Informed Decision-Making
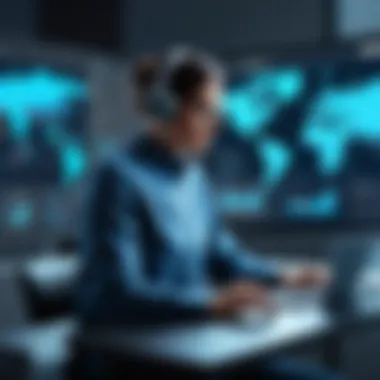
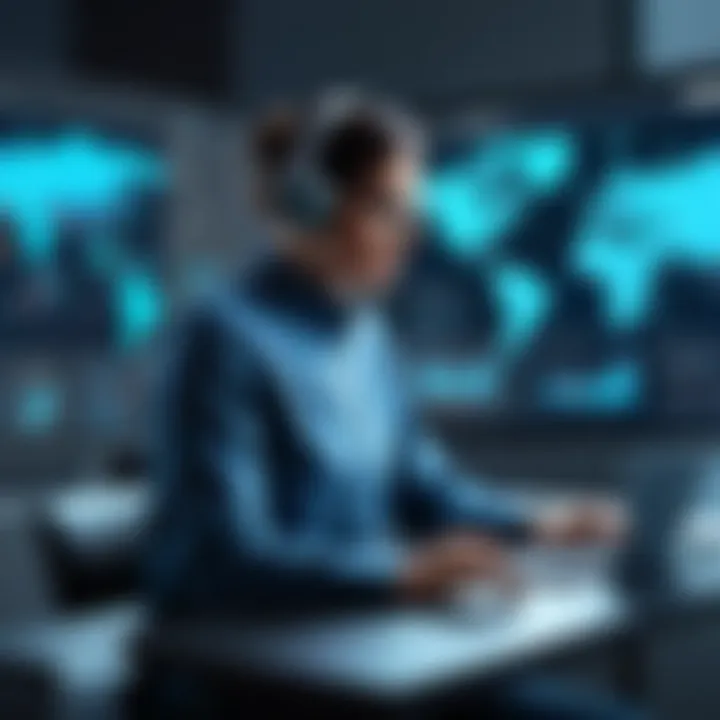
Intro
In the age of data, businesses can no longer rely solely on instinct or experience for decision-making. Instead, the integration of AI analytics tools has become a cornerstone for informed choices within organizations. These tools provide deep insights through data analysis, allowing businesses to navigate complex environments with clarity.
Data-driven decision-making is indispensable for small and medium-sized businesses that wish to optimize processes and resources. AI analytics tools empower users by streamlining operations, enhancing productivity, and supporting strategic planning. The focus of this guide is to dissect various dimensions of these tools, aimed specifically at technology decision-makers and business professionals.
As we delve deeper, we will highlight key features of AI analytics tools, explore the user experience, and identify best practices for implementation. By the end, readers will possess crucial knowledge that will aid them in making calculated, well-informed software choices.
Prolusion to AI Analytics Tools
In the contemporary landscape of business decision-making, the role of AI analytics tools is increasingly pivotal. These tools provide invaluable insights, guiding organizations to harness data effectively and make informed strategic choices. With the growing complexity of data sets and the demand for rapid analysis, AI analytics tools facilitate a deeper understanding of trends, patterns, and performance metrics.
Understanding what AI analytics tools are and how they function is the crux for any businesses aiming to leverage data for competitive advantage. These tools not only aggregate data from various sources but also apply sophisticated algorithms to generate predictive models. This capability enables businesses to anticipate market shifts and consumer behavior, thus improving their responsiveness to changing conditions.
The significance of AI analytics tools extends beyond mere data analysis. They empower small and medium enterprises to minimize guesswork in strategic decisions, enabling operators to transition from intuition-based strategies to data-driven insights. This shift is crucial in today's fast-paced ecosystem, where informed decision-making can lead to increased operational efficiency, enhanced customer experiences, and optimized resource allocation.
Moreover, the ongoing evolution of AI in analytics reflects advances in technology, encompassing increased integration capabilities, improved machine learning algorithms, and more user-friendly interfaces. As companies embark on their digital transformation journeys, the essential consideration becomes not just the adoption of these tools but ensuring they align with the organization’s objectives and existing workflows.
AI analytics tools are not just supplementary; they are integral to modern business strategies.
In summary, the introduction to AI analytics tools lays the foundation for understanding their complexities, capabilities, and importance. To leverage these tools effectively, businesses must explore their definitions, the evolution of AI in analytics, and their potential impact on businesses today.
Understanding AI Analytics Functionality
The efficiency of decision-making processes heavily relies on a deep understanding of AI analytics functionality. It allows businesses to extract actionable insights from large volumes of data. The tools used in AI analytics tackle complex data problems, offering solutions that can lead to enhanced performance and strategic advantages.
Data Collection and Integration
Data collection is the foundational step in AI analytics. The quality and relevance of data directly impact the insights generated. Proper integration of data sources ensures that organizations have a holistic view of their operations. Many businesses use a variety of systems which complicates this process.
- Importance of Effective Data Collection:
- Provides accurate data relevant to business objectives.
- Combines structured and unstructured data for thorough analysis.
To effectively collect and integrate data, organizations often utilize platforms like Microsoft Power BI, Tableau, and Google Analytics. These tools streamline the process of gathering and organizing data from multiple sources.
Predictive and Prescriptive Analytics
Predictive analytics involves using historical data to forecast future outcomes. This can be vital for decision-makers when assessing trends and making plans. Businesses can minimize risks and improve strategic planning by harnessing these capabilities.
- Benefits of Predictive Analytics:
- Informs risk management strategies.
- Enhances marketing campaigns by forecasting customer behavior.
Prescriptive analytics, on the other hand, recommends actions based on the predictions made. This functionality is critical in not just understanding what might happen, but also providing guidance on how to manage the outcomes effectively. Various companies report significant improvements in operational efficiency by utilizing these insights.
Natural Language Processing Capabilities
Natural Language Processing (NLP) is a subset of AI that enables machines to understand human language. This adds a layer of accessibility to AI analytics tools. Professionals can query data using natural language, making the tools user-friendly and efficient.
- Advantages of NLP in Analytics:
- Simplifies data interaction for non-technical users.
- Increases the speed of obtaining insights.
AI tools that employ NLP technologies empower users to derive insights without needing advanced technical skills. Such capabilities ensure wider accessibility and better engagement with analytics tools.
Understanding these functionalities is crucial for making informed decisions and optimizing workflows. Organizations need to consider the mechanisms behind these systems to effectively leverage their full potential.
Benefits of AI Analytics Tools
AI analytics tools have become integral to modern business strategies, offering numerous advantages that enhance operational efficiency and decision-making. These tools provide insights that empower organizations to utilize their data effectively. In this section, we will discuss the benefits of AI analytics tools in detail, focusing on enhanced decision-making processes, increased operational efficiency, and cost-effectiveness in software spend.
Enhanced Decision-Making Processes
One of the most significant benefits of AI analytics tools is their ability to enhance decision-making processes across various levels of an organization. Traditional data analysis methods often rely on manual inspections of data, which may lead to human errors or biases. In contrast, AI analytics tools process vast amounts of data quickly and accurately. They utilize advanced algorithms to identify patterns, trends, and insights that might be overlooked in manual evaluations.
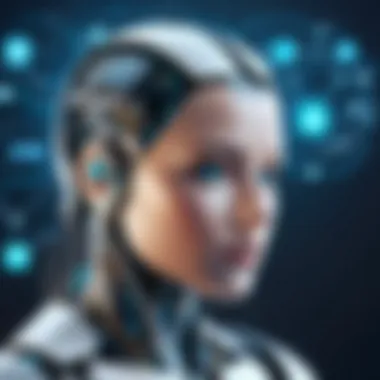
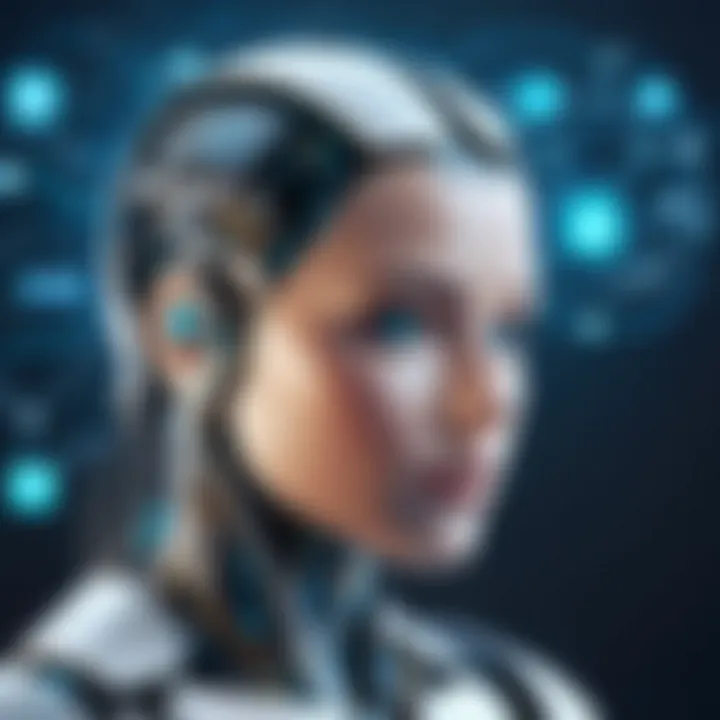
AI analytics facilitates data-driven decision-making, equipping decision-makers with actionable insights. For instance, predictive analytics can forecast future trends based on historical data. Businesses can make informed decisions about inventory management, marketing strategies, and resource allocation. This shift from intuition-based decision-making toward data-based conclusions is vital for sustaining competitiveness in today’s market.
Increased Operational Efficiency
Operational efficiency is crucial to a firm’s performance and profitability. AI analytics tools streamline operations by automating various processes that were historically labor-intensive. By integrating AI into workflows, organizations can minimize manual tasks, reduce time spent on data processing, and improve overall productivity.
For example, AI-driven data cleaning and preparation tools automatically organize and structure raw data. This automation allows analysts to focus on strategic initiatives rather than mundane tasks. Additionally, AI can optimize supply chains by analyzing real-time data to predict stock outages, ensuring timely replenishment. As a result, businesses can reduce waste and optimize their resources, leading to better operational performance.
Cost-Effectiveness in Software Spend
Investing in AI analytics tools may appear costly upfront; however, their cost-effectiveness often justifies the initial expense. By deploying these tools, organizations can achieve significant savings in the long run. For instance, AI analytics can uncover inefficiencies in operational processes and provide insights for improvement. This results in decreased operational costs.
Moreover, AI tools enable better resource management. For instance, organizations can achieve lower costs in customer acquisition by using AI analytics to segment their audiences more effectively.
"Investing in AI analytics tools can yield a substantial return on investment, reflected in reduced costs and enhanced revenue opportunities."
Ultimately, AI analytics tools provide critical advantages that bolster businesses' functions. Enhanced decision-making, increased operational efficiency, and cost-effectiveness all contribute to a strategy that positions organizations favorably in dynamically changing markets.
Challenges in Implementing AI Analytics Tools
The implementation of AI analytics tools presents various challenges that organizations must navigate to leverage their capabilities effectively. Understanding these challenges is crucial for businesses aiming to enhance their decision-making processes. Far from being a straightforward endeavor, deploying AI tools demands careful consideration of different factors, such as data privacy and security, integration with existing systems, and the skill levels of the workforce. Each of these elements plays a significant role in the successful adoption of AI analytics, which can ultimately determine the return on investment and the overall efficacy of the tools utilized.
Data Privacy and Security Concerns
Data privacy and security are paramount when adopting AI analytics tools. As organizations gather and analyze vast amounts of data, they expose themselves to potential breaches and misuse of sensitive information. The introduction of regulations such as the General Data Protection Regulation (GDPR) imposes stringent requirements on how data is collected, stored, and processed. Businesses must ensure compliance with these regulations while developing strategies that protect user data against unauthorized access.
Moreover, companies should integrate robust security measures into their analytics tools. This includes encryption, access controls, and regular audits to detect vulnerabilities. Ignoring data privacy can lead to reputational damage and significant financial penalties, making it an essential consideration during the implementation planning phase.
Integration with Existing Systems
Integrating AI analytics tools with existing systems can be a substantial challenge. Many businesses have legacy systems that may not be equipped to handle modern AI technologies. This can result in compatibility issues that complicate the data pipeline and hinder the flow of information.
To address this, organizations need a well-defined integration strategy. This often involves evaluating current systems and potentially upgrading or replacing them to ensure smooth interoperability. It might also require custom coding or APIs to bridge existing platforms with new tools. A thoughtful approach to integration prevents disruptions in service and fosters a more cohesive data environment and enhanced analytical capabilities.
Skill Gaps in the Workforce
Another significant challenge is the skill gap present in many organizations. The complexity of AI analytics tools requires trained professionals who understand both the technology and the nuance of data interpretation. Many businesses struggle to find individuals with the necessary expertise in data science, machine learning, and AI technologies.
Organizations must invest in training programs to evolve their existing workforce's skills or partner with educational institutions to cultivate talent suited for these roles. Hiring externally may offer quick wins, but nurturing talent internally ensures the long-term success of the integration process. Addressing these skill gaps fosters a more knowledgeable team that can both leverage AI tools effectively and innovate for future analytics needs.
"Addressing challenges in implementing AI analytics tools is not just an operational requirement; it is a strategic necessity that influences business outcomes."
By recognizing and tackling these challenges, businesses stand a better chance of realizing the full potential of AI analytics tools. A proactive approach can lead to improved efficiency, better decision-making, and enhanced market competitiveness.
Key Features to Consider
When exploring AI analytics tools, understanding key features is crucial. These features directly influence the effectiveness and efficiency of data analysis within an organization. As businesses look for tools that can streamline decision-making processes, they must consider various elements that can enhance their operations. Evaluating these aspects ensures that businesses choose solutions that align with their objectives and capabilities.
User-Friendliness of the Interface
A user-friendly interface is often the gateway to effective utilization of AI analytics tools. The complexity or simplicity of an interface can determine how quickly users adapt to a new system. A clear layout with intuitive navigation helps users avoid frustration, which can result in delays or improper usage of the tool. Moreover, when a tool is easy to use, it reduces the learning curve involved for team members. Consequently, this can lead to improved adoption rates within the organization.
Furthermore, a well-designed interface can enhance collaboration among teams. When the interface is straightforward, it facilitates better communication of insights derived from data analysis. All members can participate in discussions without needing extensive training on the tool itself. In this way, the user-friendliness of the interface emerges as a critical feature to assess when selecting AI analytics tools.
Scalability of the Tool
Scalability refers to the ability of a tool to grow alongside a business's needs. As organizations evolve, their analytical requirements may change. If a tool is not scalable, it may become obsolete as a company increases its data volume or complexity of analyses. Therefore, it is essential to consider how a tool can adapt to such changes.
A scalable tool not only accommodates expanding data environments but also allows for the integration of new features without disrupting existing infrastructures. This adaptability ensures that businesses do not need to frequently switch tools as they grow, which can be costly and disruptive. Thus, scalability should be a fundamental consideration when evaluating AI analytics tools.
Reporting and Visualization Options
Effective reporting and visualization capabilities are paramount for translating complex data insights into understandable formats. Tools that provide robust reporting options enable users to generate detailed analyses and summaries effortlessly. This functionality assists decision-makers in grasping critical patterns and trends that may influence their strategies.
Moreover, visualization options enhance comprehension by presenting data in graphical formats. Graphs, charts, and heatmaps can illustrate information that would otherwise be convoluted in raw data tables. The clarity brought by effective visualization aids in quicker decision-making by highlighting essential insights without overwhelming users with data.
"In an era of vast data, making sense of that data with well-designed tools is not just advantageous; it is necessary for strategic growth."
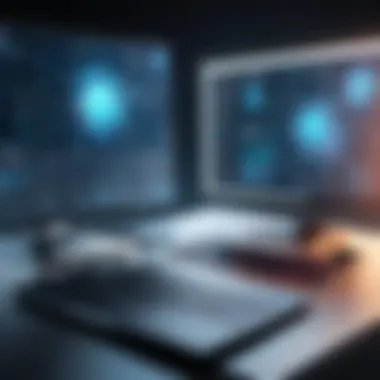
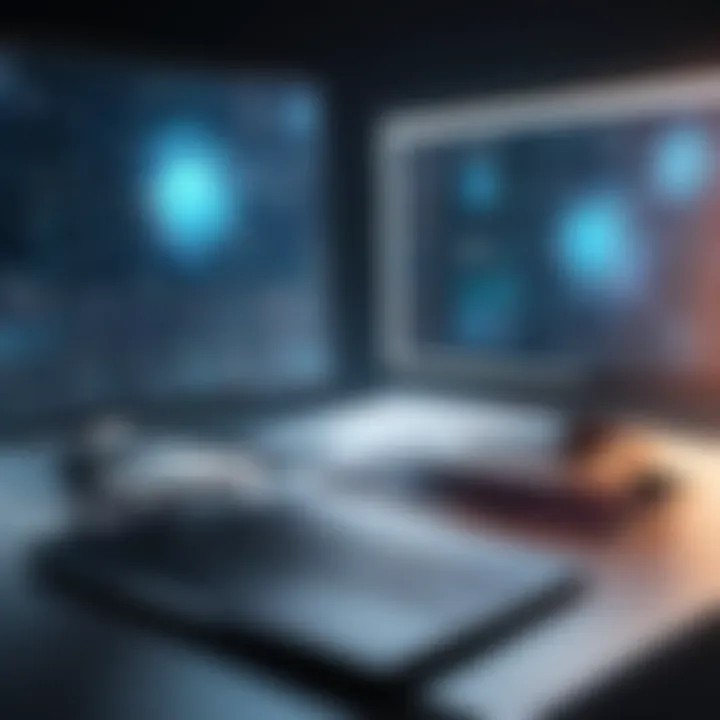
By carefully assessing these aspects, businesses can select tools that empower their teams and enhance their decision-making processes.
AI Analytics Tools for Different Industries
The integration of AI analytics tools across various industries holds significant relevance in today’s data-driven landscape. By utilizing these tools, organizations can harness vast amounts of data to extract insights that facilitate informed decision-making. Each industry exhibits unique characteristics and challenges that can be addressed through tailored AI solutions. This section examines the applications of AI analytics tools in three key sectors: healthcare, retail, and financial services, shedding light on their benefits and essential considerations.
Healthcare and AI Analytics
AI analytics tools are transforming the healthcare industry by improving patient outcomes and operational efficiencies. For instance, these tools can analyze patient data, enabling healthcare providers to identify trends and develop personalized treatment plans. They aid in the early diagnosis of diseases by utilizing predictive analytics to evaluate historical medical records and real-time health data.
Moreover, AI can streamline administrative tasks, such as patient scheduling and billing, allowing healthcare professionals to focus more on patient care rather than paperwork. The implementation of AI also enhances drug discovery processes, making them faster and more cost-effective. As data privacy is paramount, healthcare organizations must prioritize compliance with regulations like HIPAA to protect sensitive information.
Key considerations include:
- Ensuring data integration from various sources
- Training staff on the use of AI tools
- Constantly monitoring performance metrics to improve outcomes
Retail and Consumer Insights
In the retail sector, AI analytics tools play a critical role in shaping customer experiences and optimizing inventory management. By analyzing consumer behavior across multiple touchpoints, retailers gain valuable insights into purchasing patterns and preferences. This data can then inform marketing strategies and promotional campaigns, thereby increasing customer engagement.
AI-driven tools can also forecast demand, helping retailers maintain optimal inventory levels and reduce excess stock. This leads to cost savings and better resource allocation. Additionally, personalized recommendations powered by AI improve the shopping experience, driving customer loyalty and repeat sales.
Important elements to consider are:
- Data security to protect customer information
- Continual refinement of algorithms based on consumer feedback
- Assessing the effectiveness of targeted marketing campaigns
Financial Services and Risk Management
Financial services benefit immensely from AI analytics tools, particularly in areas like fraud detection and risk assessment. By leveraging advanced algorithms, institutions can analyze transaction data in real-time, identifying anomalies that may indicate fraudulent activities. This enhances security and builds trust with consumers.
Furthermore, AI analytics aids in credit scoring and risk modeling. Financial professionals can utilize historical data to predict potential defaults and inform lending decisions. This capability not only reduces risk but also promotes responsible lending practices. As with other sectors, the integration of AI must be approached with caution, considering regulations like GDPR that govern data usage.
Considerations for this industry include:
- Risk mitigation strategies related to algorithm biases
- Compliance with financial regulations
- Investment in training personnel to adapt to AI technologies
The strategic use of AI analytics tools in these industries exemplifies the potential for improved decision-making and operational efficiencies. Each sector may face different challenges, but the benefits of adopting these tools are evident in their capacity to enhance performance and drive innovation.
Case Studies: Successful Implementation
Case studies serve as powerful narratives that illustrate how AI analytics tools can manifest tangible benefits in real-world scenarios. These examples not only showcase the successful integration of technology but also provide insight into practical applications and best practices that other businesses can adopt. By examining specific instances, organizations can understand key challenges faced and overcome, ensuring a smoother implementation process when selecting and deploying AI analytics tools. This section highlights how different industries leveraged AI analytics to improve decision-making and operational efficiency, thus making it an essential discussion point in the larger context of informed decision-making in business.
Case Study in Retail
In the retail sector, a notable case study comes from Walmart, which has implemented AI analytics tools to enhance inventory management and customer experience. The retail giant has integrated predictive analytics to enhance its supply chain operations. By analyzing purchasing trends and consumer behavior patterns, Walmart can forecast demand more accurately, reducing overstock and stockouts.
A significant aspect of this implementation involved the handling of vast amounts of data from various sources like point-of-sale systems, loyalty programs, and online interactions. This integration allowed for real-time data analysis, facilitating immediate responsiveness to market changes.
The results were compelling. Walmart saw a significant reduction in excess inventory and improved customer satisfaction. This case illustrates the broader implications of AI analytics in optimizing operational efficiency and provides valuable lessons about data integration and response time in retail management.
"The ability to predict consumer behavior in real-time has transformed our approach to inventory. AI is not just a tool; it’s a strategic advantage." - Walmart Supply Chain Director
Case Study in Healthcare
In healthcare, the Mount Sinai Health System has made impressive strides by utilizing AI-driven analytics tools for patient care optimization. The system employs predictive analytics to assess patient risks and needs, which plays a critical role in managing chronic diseases.
Mount Sinai harnesses data from electronic health records (EHRs) to identify patients who may require intervention or closer monitoring. This proactive approach has enabled healthcare providers to target resources more effectively, thereby improving patient outcomes and reducing hospital readmission rates.
The results were quite positive. A study indicated that the hospital saw a 15% reduction in readmissions among patients diagnosed with heart failure within the first year of implementation. This case study showcases how healthcare facilities can leverage AI analytics to transition from reactive to proactive patient management, indicating a broader shift needed in the industry.
Best Practices for Selecting AI Analytics Tools
The selection of AI analytics tools can make or break an organization's decision-making capabilities. Implementing the right tool enables companies to harness data effectively, helping to optimize processes and streamline operations. Conversely, choosing an unsuitable analytics tool can lead to wasted resources and misguided strategies. To mitigate these risks, it is advisable to follow best practices that can guide organizations through the selection process.
Conducting a Needs Assessment
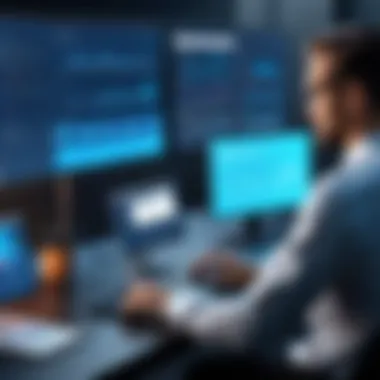
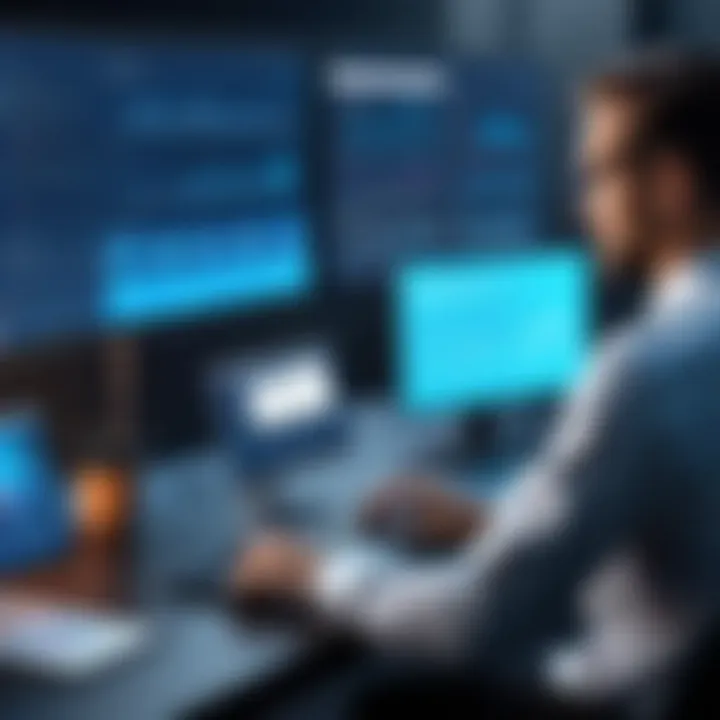
Before diving into the selection of a particular AI analytics tool, understanding the specific needs of the organization is crucial. A needs assessment allows stakeholders to identify what they intend to achieve through the use of analytics. It helps to clarify clear objectives.
This phase can include:
- Identifying Key Performance Indicators (KPIs): Outline which metrics matter most to your business.
- Involving Stakeholders: Gather input from various departments to understand their data requirements.
- Defining Scope: Determine the scale and range of data analyses needed.
By performing a comprehensive needs assessment, organizations can avoid investing in features that do not align with their goals, leading to better-targeted outcomes.
Evaluating Vendor Credibility
Once the needs are clearly defined, attention must turn to potential vendors. Evaluating vendor credibility is essential, as it can significantly affect the success of the implementation. Key considerations should include:
- Reputation in the Market: Research the vendor’s track record and customer feedback.
- Technical Support and Training: Assess the kind of support and resources the vendor offers for onboarding and ongoing assistance.
- Performance Metrics: Look at case studies detailing how other companies have benefited from the tool.
This evaluation helps ensure that the selected vendor is reliable, reducing the risks associated with poor support or inadequate functionality.
Pilot Testing and Feedback Incorporation
Finally, trialing the tool before full implementation is a best practice often overlooked. Pilot testing allows organizations to assess the real-world application and efficiency of the chosen AI analytics tool within their environment.
During this process:
- Collect User Feedback: Engage users to gather their insights and experiences.
- Monitor Performance: Measure how well the tool meets the established objectives from the needs assessment phase.
- Make Adjustments: Be open to making necessary changes based on feedback; iteration is key for improvement.
Through pilot testing, organizations can significantly refine their selection process, ensuring they choose a tool that fits their needs and enhances their operational capabilities effectively.
Effective best practices in selecting AI analytics tools correlate directly with successful business outcomes. By carefully conducting a needs assessment, evaluating vendor credibility, and embracing pilot testing, companies can maximize benefits and minimize risks.
Future Trends in AI Analytics Tools
AI analytics tools are continually evolving, responding to the rapidly changing landscape of technology and business needs. Understanding the future trends in this domain is crucial for organizations seeking to leverage data effectively for decision-making. This section discusses important emerging trends, like no-code solutions and increased automation in AI analytics tools, clarifying their implications for businesses.
Emergence of No-Code Solutions
No-code solutions are gaining traction in the AI analytics world due to their ability to democratize technology. These tools allow users, regardless of their technical expertise, to create data analytics workflows and dashboards. The appeal lies in the simplicity and speed these platforms offer. Companies are discovering that these solutions enable quicker data-driven decision-making.
Some key benefits of no-code solutions include:
- Accessibility: They empower non-technical users to interact with data meaningfully.
- Cost Efficiency: Organizations can reduce reliance on specialized IT personnel for every analytic task, freeing up resources for more strategic initiatives.
- Rapid Deployment: Businesses can quickly implement changes and test analytics insights without prolonged development cycles.
As more businesses adapt to these no-code platforms, it may reshape workforce dynamics. Business users feel more confident in their abilities, leading to enhanced innovation and agility in responses to market demands.
Increased Focus on Automation
Automation in AI analytics tools is transforming how organizations handle data processing and analysis. As businesses continue to generate massive amounts of data daily, automating mundane tasks becomes necessary to ensure efficiency and accuracy. Automation helps in generating insights faster, facilitating timely decision-making processes.
Considerations around automation include:
- Enhanced Data Processing: Automated tools can streamline data cleaning and preparation efforts, allowing analysts to focus on interpretation and strategy.
- Predictive Analytics: Automation enhances forecasting capabilities, harnessing algorithms to quickly identify trends and anomalies in vast datasets.
- Continuous Learning: Many automated systems can learn from new data inputs over time, improving their prediction accuracy.
As businesses embrace these automated AI analytics solutions, staying updated with the latest advancements is key. Continuously integrating these tools allows organizations to remain competitive and proactive in their strategic planning.
"The future of analytics lies in tools that empower users at all levels to derive insights swiftly and accurately, driving real-time decision-making across the board."
Epilogue
In the rapidly evolving landscape of business technology, the significance of AI analytics tools cannot be overstated. This article has delved into various aspects of these tools, emphasizing their role in facilitating informed decision-making. AI analytics tools empower organizations by providing actionable insights derived from complex data sets. As these tools enhance the quality and speed of decision-making, the relevance of integrating them into business strategies becomes increasingly apparent.
Summary of Key Insights
Throughout the exploration of AI analytics tools, several key insights have emerged:
- The capabilities of these tools encompass data collection, predictive analytics, and natural language processing, each contributing to understanding business trends.
- There are notable benefits such as improved operational efficiency, enhanced decision-making processes, and overall cost-effectiveness.
- However, challenges exist. Issues related to data privacy and security, integration with current systems, and workforce skill gaps must be addressed thoroughly.
- Key features for selection include user-friendliness, scalability, and robust reporting options. These criteria help ensure that businesses select tools that fit their specific needs.
- Different industries, such as healthcare and finance, have tailored applications for AI analytics, showcasing versatility across sectors.
"Investing in AI analytics is not just about technology; it’s about transforming how businesses perceive and act on data."
Final Recommendations for Businesses
When considering AI analytics tools, businesses should adopt a strategic approach:
- Conduct a Needs Assessment: Identify the specific needs of your organization. Understand what challenges you aim to resolve through analytics.
- Evaluate Vendor Credibility: Research potential vendors thoroughly. Look at their market presence, client testimonials, and case studies to determine reliability.
- Pilot Testing and Feedback Incorporation: Before full-scale implementation, conduct pilot testing to assess functionality. Collect feedback from users to optimize the tool's integration.
- Stay Current with Trends: The landscape of AI analytics is dynamic. Keep abreast of emerging technologies and trends to ensure tools remain effective and competitive.
By following these recommendations, organizations can effectively harness the power of AI analytics tools, thus positioning themselves for sustained growth and innovation.