Top Tools for Effective Big Data Analysis
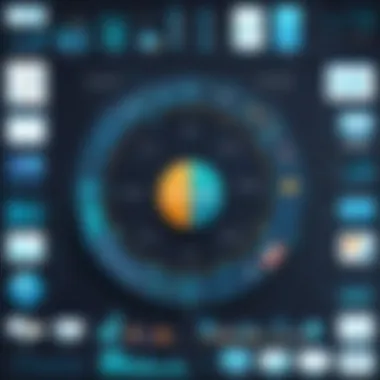
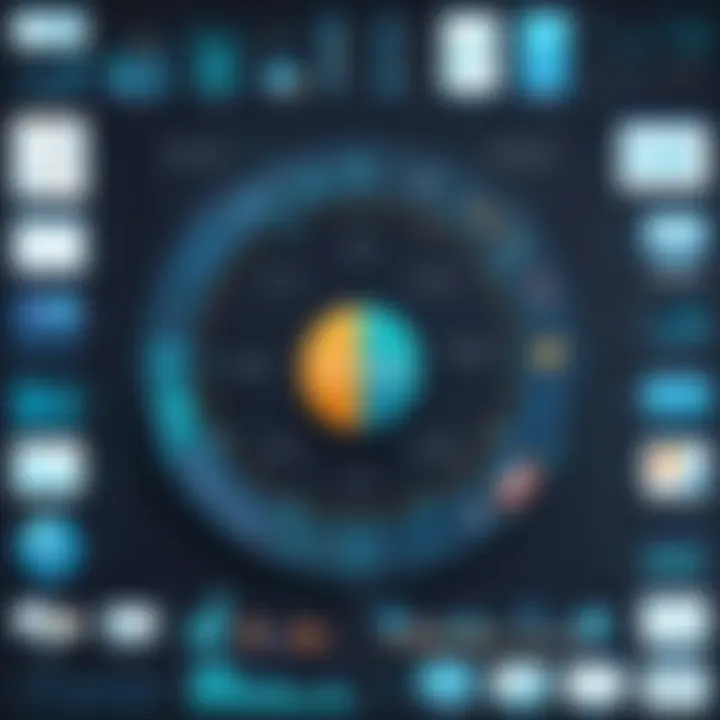
Intro
In an age where data drives decision-making, the ability to analyze and interpret vast amounts of information is crucial for businesses of all sizes. As small and medium-sized companies venture into the realm of big data, they often encounter the challenge of selecting the right tools to handle this complex process. The landscape is flooded with options, each boasting its own set of features, strengths, and weaknesses. This comprehensive overview aims to shine a light on the essential tools, equipping novices and seasoned professionals alike with insights to navigate these waters effectively.
Understanding big data doesn't just mean being able to process numbers and figures; it requires grasping the underlying technology, the nuances of different software, and how they can be applied to real-world questions. This article breaks down the various categories of data analysis tools, exploring their essential capabilities and showcasing unique features that set them apart. By the end, you should feel confident in your ability to select a tool tailored for your specific business needs.
Overview of Key Features
Big data analysis tools come equipped with a myriad of capabilities designed to transform raw data into actionable insights. Here’s what to look for:
Essential Software Capabilities
- Data Integration: The ability to connect with various data sources (e.g., CRM systems, databases, cloud storage) is fundamental. A good tool should enable seamless data aggregation.
- Analytics Provisions: Whether it's statistical analysis, predictive modeling, or machine learning algorithms, the tool must provide diverse analytical capabilities to cater to different business needs.
- Visualization Functions: Data is best understood when presented visually. Look for tools that offer robust visualization features to create comprehensive dashboards, charts, and graphs.
- Scalability: As your data grows, so should your tools. Ensure the software can handle increasing volumes without a hitch.
- Security Features: Protecting sensitive data is non-negotiable. Features like data encryption and user permissions are essential to safeguard your information.
Unique Features That Differentiate Options
Not all tools are created equal. Here are some distinguishing characteristics that may influence your choice:
- Real-time Analytics: Some platforms excel at offering real-time insights, essential for businesses that require immediate answers.
- Machine Learning Integration: Tools equipped with machine learning capabilities can automate and enhance analysis, allowing for smarter predictions and insights.
- User Customization: The ability to tailor dashboards and reports according to specific user regulations or preferences adds significant value.
- Collaboration Tools: Features designed for team collaboration can enhance the overall utility of a platform, allowing teams to work together, share insights, and track progress.
- Cost-Effectiveness: Budget constraints are common for SMBs. Evaluating the pricing structures—especially whether there’s a free tier or trial period—can be a game-changer in your decision process.
User Experience
Beyond functionality, the user experience of a tool is paramount to its overall effectiveness.
Interface and Usability
A cluttered interface can easily overwhelm users, especially those who are not well-versed in technical jargon. Look for a clean, intuitive layout that simplifies navigation. Tools should be straightforward enough that your team can start crafting reports without extensive training.
Support and Community Resources
It's crucial for users to have access to support channels. Whether it’s tutorials, webinars, or active community forums on platforms like Reddit or Facebook, comprehensive support can make the difference between a successful implementation and a frustrating experience. Having easy access to documentation and learning resources can reduce the learning curve when integrating new tools into daily operations.
"The right analytical tool can turn a mountain of data into insights that steer business strategies toward success."
Understanding and analyzing big data demands appropriate tools—tools that not only fulfill technical needs but also provide an enjoyable user experience. Keeping an eye on both aspects ensures your business harnesses the full potential of big data analytics.
Prologue to Big Data Analysis
As we step into an era where data reigns supreme, understanding the intricacies of Big Data analysis becomes paramount. This section sets the stage for the subsequent chapters by elucidating what Big Data entails and why analyzing it is crucial for businesses today.
Big Data is not just a buzzword; it acts as a cornerstone for strategic decision-making across various industries, reshaping how companies operate. By unlocking insights hidden within vast datasets, organizations can let data drive their actions, rather than relying solely on intuition. In effect, it allows businesses to tailor their products and services to meet customer expectations more effectively, leading to heightened satisfaction and loyalty.
Defining Big Data
Big Data encompasses massive volumes of structured and unstructured data generated from various sources. Think of it as a large ocean filled with information from social media interactions, sensor data, online transactions, and more. The term itself highlights three critical characteristics, often referred to as the "Three Vs": Volume, Variety, and Velocity.
- Volume refers to the sheer amount of data that is produced daily, which runs into zettabytes.
- Variety addresses the different forms data can take—from text and images to audio and video.
- Finally, Velocity speaks to the speed at which this data is being generated and is required to be processed.
With these dimensions in mind, it’s salient to recognize that managing and analyzing such colossal datasets come with its own set of challenges.
Importance of Data Analysis
The importance of data analysis in the Big Data landscape cannot be overstated. It serves as the roadmap to deriving meaningful patterns and actionable insights from data. Companies that harness effective analytical tools can make informed decisions, anticipate market trends, and gain a competitive edge.
"In data analysis, context is king."
Data analysis allows organizations to:
- Mitigate Risks: When companies can analyze historical data, they can identify potential pitfalls and strategize accordingly.
- Enhance Efficiency: By understanding patterns, businesses can streamline operations and reduce redundancies.
- Boost Innovation: Data analysis enables insightful predictions about future consumer behavior, spurring innovative product development.
The critical takeaway here is that without effective data analysis, the monumental effort put into collecting and storing data could go to waste. Only through analysis can businesses translate raw data into valuable information that drives growth.
Key Characteristics of Big Data Tools
In the fast-evolving realm of big data, organizations are increasingly relying on specific tools that can harvest value from enormous datasets. It's not merely about handling metrics; it's about effectively transforming raw data into actionable insights. The key characteristics of big data tools serve as the backbone to achieving this goal. Understanding and emphasizing these characteristics allows businesses to select optimal tools that align with their needs.
Scalability
When it comes to big data, scalability stands tall as a foundational characteristic. In practical terms, a scalable tool can handle increased workload without a hitch. As data inflow expands—whether it's from user interactions, sales transactions, or IoT devices—organizations must ensure their chosen tools can grow alongside their needs.
Consider a retail company gearing up for the holiday season. Anticipating a spike in online transactions, they would require tools that can handle colossal volumes of customer behaviour data on the fly. If the tool is not scalable, it can lead to crashes or lag, which could directly impact revenue and customer experience.
Moreover, scalability isn't just about accommodating more data; it's also about integrating additional features as required. For example, tools that support a plug-and-play model allow businesses to deploy new capabilities as they evolve. This flexibility can be a game-changer.
Speed of Processing
The speed of processing is another non-negotiable trait of big data tools. In specific industries such as finance, where real-time data processing is essential for risk assessment and decision-making, this characteristic can influence outcomes tremendously. Tools that provide quick insights can propel businesses ahead of the competition, allowing them to react promptly to market changes.
For instance, think about a logistics company monitoring the location of its delivery vehicles in real time. The ability to gather, process, and visualize this data swiftly enables more efficient routing, ultimately saving time and reducing operational costs. A tool that lags can quickly undermine such advantages, resulting in lost opportunities and potentially high costs.
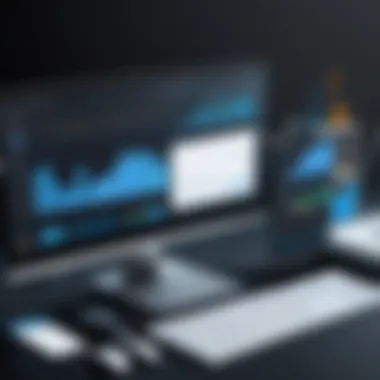
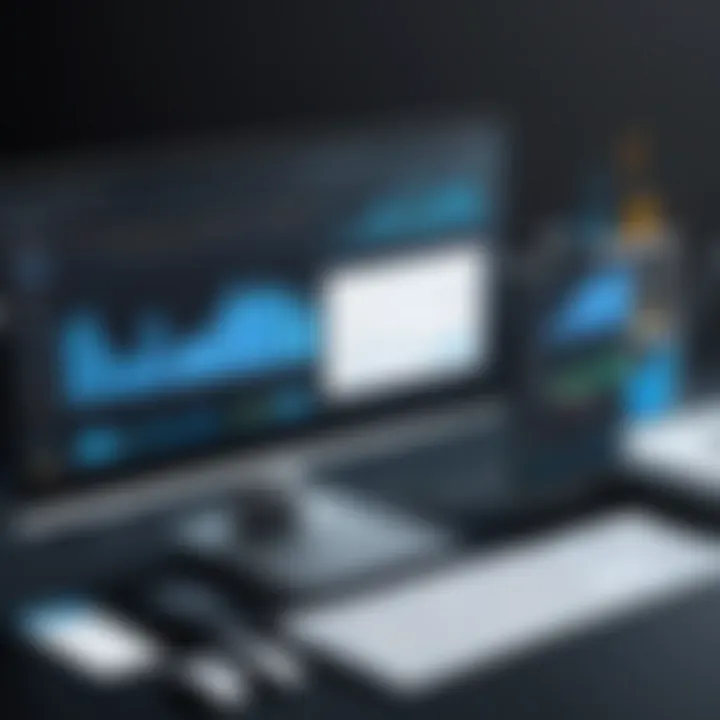
It is vital to note the significance of the underlying architecture in facilitating speed. Tools that employ in-memory computing, for instance, are designed to process data as it’s needed rather than relying on disk storage for retrieval, resulting in a marked improvement in speed.
Data Variety
In today’s data-driven world, data variety denotes the capability of tools to handle diverse data types from various sources. This characteristic is particularly crucial, as data can come in structured formats like databases or unstructured forms such as text and images. To achieve comprehensive analytics, tools must adeptly manage this variety.
For example, consider a marketing team analyzing customer sentiment. Integrating data from surveys, social media comments, and purchase history helps create a richer picture. Tools that can seamlessly combine these data types eliminate silos, allowing for more nuanced insights.
In addition, accommodating various data formats challenges traditional relational databases, necessitating more versatile solutions, like NoSQL databases or data lakes. These more advanced tools enable businesses to evolve their data strategies without extensive reconfiguration.
Tools that embrace data variety help organizations escape from the rut of narrow insights, facilitating a broader perspective on trends and analytics.
To sum up, understanding the key characteristics of big data tools not only facilitates better selection and alignment with business needs but also highlights the potential benefits when these tools are employed effectively. These characteristics—scalability, speed of processing, and data variety—ensure that organizations are not just collecting data but actively transforming it into a strategic asset.
Types of Big Data Analysis Tools
Understanding the different types of big data analysis tools is pivotal to effectively navigating the vast landscape of data analytics. Various tools fulfill unique functions, each playing an integral role in transforming raw data into valuable insights. In today’s digital age, where data is akin to oil, identifying the right tool is essential for businesses aiming to stay ahead of the curve.
The importance of categorizing these tools lies in the diverse requirements of businesses and how they utilize data. Below, we delve into several main categories of tools that stand at the forefront of big data analysis:
Statistical Analysis Tools
Statistical analysis tools are fundamentally designed to help users sift through vast data sets to derive meaningful statistics. They provide capabilities to conduct quantitative analysis, allowing data analysts to perform methodical calculations which inform decision-making.
Some key benefits include:
- Robust Data Interpretation: These tools help determine patterns, showing shifts or anomalies that may not be visible at first glance.
- Hypothesis Testing: Users can test theories against data to confirm or deny assumptions, enhancing the reliability of conclusions drawn.
- Predictive Analytics: Utilizing past data, statistical tools can help forecast trends, which is critical in fields like finance and marketing.
Examples of statistical analysis tools are SPSS and R, both notorious for their comprehensive capabilities in tackling complex analyses. For anyone working with significant datasets, mastering these tools is not just beneficial; it’s indispensable.
Machine Learning Tools
Machine learning tools represent a more automated approach to big data analysis. Unlike traditional analytical tools that rely heavily on human intervention, machine learning tools use algorithms that learn from data, automatically improving their performance over time.
Consider these advantages:
- Automation of Insights: Users can automate data patterns recognition, streamlining the analysis process.
- Scalability: As data grows, machine learning models can adapt without requiring substantial reprogramming, providing continuous insights for evolving datasets.
- Personalization: These tools excel in customizing user experiences, making them particularly valuable for marketing, product recommendations, and content delivery strategies.
TensorFlow and scikit-learn are examples of machine learning frameworks popular among developers and data scientists alike. Their ability to create predictive models makes them a game-changer in customer-centric industries.
Data Visualization Tools
Data visualization tools serve to present large datasets in a more digestible format. By translating complex data into graphical representations, they enable decision-makers to grasp insights swiftly. This visual storytelling is more intuitive than numbers alone.
Key benefits of employing data visualization tools include:
- Clarity: Visuals simplify the data narrative, helping users identify key trends and insights at a glance.
- Engagement: Good visuals can captivate an audience, whether in a corporate presentation or an analytical report.
- Real-Time Monitoring: Many of these tools allow for live data feeds, enabling organizations to make decisions based on the most current information.
Prominent data visualization tools like Tableau and Power BI empower businesses to communicate findings efficiently through interactive dashboards and charts. They turn data into stories that evoke understanding and action.
ETL Tools
Extract, Transform, Load (ETL) tools are specialized for data integration. They enable organizations to gather data from various sources, clean it, and load it into a central storage solution (like a data warehouse). This is vital for ensuring that data analysts have access to high-quality, consistent data.
Advantages of ETL tools include:
- Data Quality: ETL processes help in cleaning and transforming data, making it reliable for analysis.
- Centralization: They consolidate various data sources, providing a single point of access for analytics.
- Efficiency: Automating this process saves time and reduces labor costs associated with manual data handling.
Popular ETL solutions like Apache Nifi and Talend are favored for their robust data processing capabilities, enabling analysts and businesses to focus on data interpretation rather than data prep.
Popular Big Data Tools Overview
Big data tools are the backbone of modern data analysis, playing a crucial role in transforming raw data into actionable insights. In this fast-paced digital environment, organizations can no longer afford to ignore the gigabytes and terabytes of information they generate. Understanding the tools available not only helps in optimizing data handling but also enables businesses to leverage their data for strategic advantages. Investing time in knowing these tools is essential for making informed choices, especially for tech-savvy individuals and business professionals aiming to be ahead of the curve.
Apache Hadoop
Apache Hadoop is akin to the Swiss Army knife of big data tools. It’s an open-source framework that allows for distributed storage and processing of large data sets using simple programming models. The beauty of Hadoop lies in its ability to scale out across hundreds or thousands of servers. It’s like having a vast team of workers who can simultaneously tackle raw data in chunks, making it suitable for enterprises that handle enormous volumes of information.
That said, Hadoop is not without its quirks. The learning curve can be steep for newcomers, and while it offers flexibility, the complexities involved can sometimes deter businesses from employing it. However, its strong community support provides resources for troubleshooting, and its versatility in various industries makes it a staple in data analysis.
Apache Spark
Where Hadoop might be great for large-scale data processing, Apache Spark steps in as the speed demon of the big data arena. Spark is designed for high-speed data processing and can handle streaming data in real-time. In settings where immediate decision-making is crucial—think financial markets or emergency response systems—Spark shines.
Spark's in-memory processing is a game changer. It can speed up applications by orders of magnitude, processing large amounts of data much faster than Hadoop's MapReduce, due to its ability to retrieve data from memory rather than disk. However, doing so requires more memory resources, which could mean higher upfront costs for businesses.
Tableau
Tableau is not just a data analysis tool but rather a visual storyteller. With its user-friendly interface, it empowers users—from analysts to executives—to create visually appealing and interactive dashboards. In a world where visuals often communicate more than numbers, Tableau provides an intuitive platform to translate complex data into understandable formats.
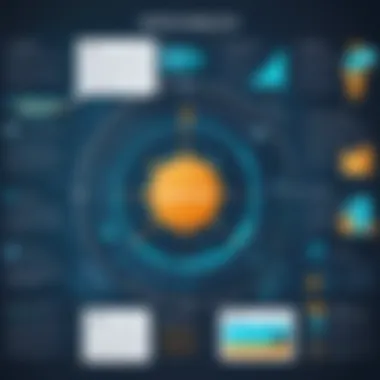
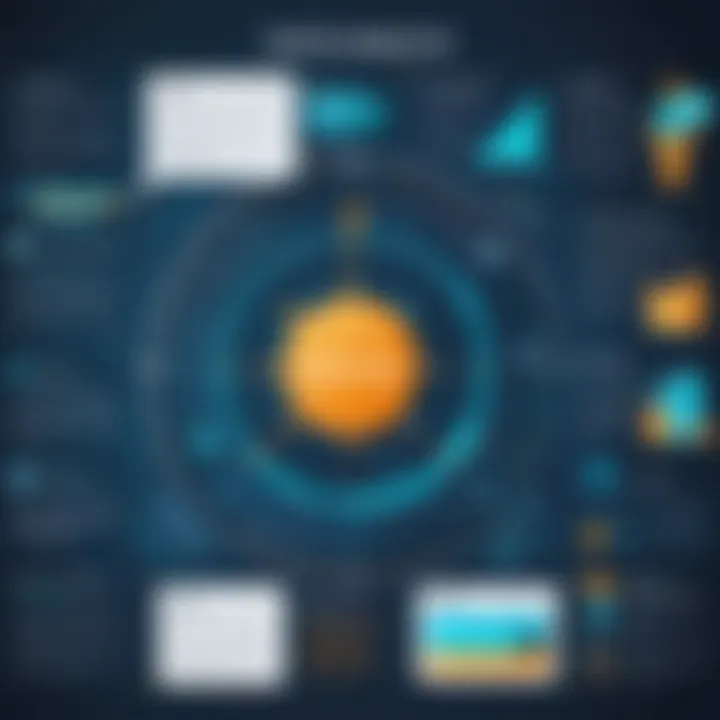
One of the strongest features is its ability to connect seamlessly with multiple data sources, be it traditional databases or big data environments like Hadoop or Spark. Clarity in presentation is its forte, reducing the jargon-heavy discussions into clear narratives that stakeholders can quickly digest. However, some users may find that while it excels in visualization, in-depth statistical analysis could be limited compared to other tools.
Power BI
Microsoft's Power BI positions itself as a comprehensive suite for business analytics. The tool allows users to transform raw data into meaningful insights through an easy-to-navigate interface, making it an appealing option for those who may not have extensive technical backgrounds. Its capabilities range from data preparation to insight sharing, backing organizations with the tools to make data-driven decisions.
Power BI integrates well with Microsoft’s suite of applications, placing it in a favorable position for businesses already invested in Microsoft technologies. However, just like any other tool, it has its peculiarities. For larger datasets or highly complex analyses, performance can lag, necessitating a balance between ease of use and depth of functionality.
"Choosing the right tool is just as critical as the analysis itself."
Ending
By familiarizing themselves with tools like Apache Hadoop, Apache Spark, Tableau, and Power BI, organizations can find the right fit based on their unique needs and constraints. Each tool offers distinct advantages and challenges, and understanding these points will guide informed decisions in the intricate landscape of big data analysis.
Criteria for Choosing the Right Tool
Choosing the right tool for big data analysis isn’t just a shot in the dark; it’s a nuanced decision that can have profound implications for any organization. The implications span from operational efficiency to actionable insights gleaned from the data. With a myriad of tools available in today’s marketplace, honing in on what best suits your specific needs can feel a bit overwhelming. However, there are key criteria that simplify this daunting task.
Assessing Business Needs
Determining the requirements of your business is like gathering your ducks in a row before making an important purchase. Not every tool is a one-size-fits-all, and understanding your unique objectives is paramount. Start by identifying the specific problems you aim to address with your data. Do you want to improve customer engagement? Enhance supply chain efficiency? The clearer you are about your goals, the better you can evaluate tools that align with those aims.
Once goals are set, consider the volume, velocity, and variety of your data. Not all tools are capable of handling massive datasets efficiently. For instance, if your business handles real-time data streams, you might want to focus on tools that are adept at processing this kind of data with minimal latency. This thoughtful assessment ensures you're not just picking the shiniest tool off the shelf.
Budget Considerations
When you think of big data tools, it's easy to picture costly investments, but being practical about your budget can save a lot of headaches down the line. Different tools come with varying price tags, depending on their functionality and the scale at which they operate. Be careful, though; the cheapest option may not always provide the best value.
It's essential to weigh the total cost of ownership, which includes upfront costs, maintenance, and any additional features you might need down the road. Companies like Tableau and Power BI, for example, have different pricing structures depending on the scale and features utilized. Keeping your financial constraints in mind can help narrow down your options without breaking the bank.
The cost of a tool is not just about the initial outlay; it’s about the total value it returns over its life cycle.
Ease of Use
Ultimately, regardless of how powerful a tool may be, if it's as user-friendly as a Rubik’s Cube blindfolded, it’s not gonna hold much appeal. The ease of use is critical for successful adoption in any organization. If the learning curve is steeper than a mountain, employees might resist using the tool, which defeats the purpose.
Evaluate whether the tool offers a straightforward user interface, adequate training resources, and support. Tools like Apache Spark can be highly capable, but they may require a certain skill level to utilize effectively. On the flip side, user-friendly interfaces such as those in Power BI or Tableau might make life easier for your team and streamline the analysis process significantly.
Thus, when navigating your choices, consider not only the capabilities of a tool but also how accessible it is for your team. After all, a tool can only be as effective as the people using it.
Integration with Other Systems
Integrating big data tools with existing systems is an often overlooked but critical aspect for organizations aiming to harvest the full potential of their data. When tools can smoothly communicate with other systems, it paves the way for efficient workflows and a more cohesive strategy in data management and application. Essentially, it's about ensuring the sum of parts is greater than just the individual whole.
Having tools that can integrate effectively means a business can utilize data from various sources, allowing for a more holistic analysis. This can lead to actionable insights across departments, enhancing decision-making and enabling a quick response to market changes. Whether it's a CRM system talking to an analytics platform or an ETL tool feeding data into a dashboard, these integrations are crucial.
APIs and Data Compatibility
One of the linchpins in successful integration is the availability of Application Programming Interfaces (APIs). They serve as the bridge that connects disparate systems—enabling them to communicate and share data seamlessly. From a technical viewpoint, well-documented APIs can save organizations a good chunk of headaches down the line. Data compatibility is also vital; it isn't merely about having an API but ensuring that the format of data—for example, CSV, JSON, or XML—meets the requirements of the receiving tool.
Consider how a retail business uses a customer relationship management software. By integrating it with a data analysis tool through an API, they can track customer behavior and preferences in real-time. If data formats don't align, however, then even the best tools can become a hot mess—leading to delays in insight. The importance lies not just in having a connection but ensuring that it works fluidly and efficiently.
Collaboration Features
In today’s fast-paced business environment, collaboration features in big data tools can no longer be seen as optional. The ability to share insights and findings in real-time can make or break decision-making processes. Collaboration goes beyond simply sharing access; features should foster a conducive environment for teamwork. This might include functionalities like version control, annotations for shared datasets, and the ability to annotate or chat within data visualization interfaces.
With strong collaboration features, teams can work through complex datasets together, allowing diverse perspectives to merge. This can often lead to more nuanced insights. Imagine a finance team and marketing team working simultaneously on customer data. If one team discovers a trend, they can instantly communicate with the other, enabling a swift pivot in strategy that would have taken far longer without integrated collaboration tools.
"Integrated systems are crucial for making informed, timely decisions in today’s complex data landscape."
Having systems that allow collaboration ensures everyone is on the same page and hopping in the wagon together. As more teams begin to work remotely or in diverse locations, the importance of these features rises exponentially. Integrating tools with strong collaborative capabilities leads directly to enhanced productivity and more informed decisions.
Real-World Applications of Big Data Tools
Big data tools are not just abstract concepts floating in the tech cloud; they find their true essence when applied in real-world contexts. These applications highlight their significance across various industries, underscoring the transformative power of data harnessed for practical purposes. This section will illustrate how different sectors leverage big data tools to glean insights, drive decisions, and enhance overall efficiency.
Healthcare Industry
In healthcare, big data analysis tools are paving the way for personalized medicine and improved patient outcomes. With the abundance of patient data available, organizations can analyze patterns that may not be visible through traditional means. For instance, hospitals use tools like Apache Spark to analyze vast datasets from electronic health records. This enables them to identify risk factors, predict disease outbreaks, or even personalize treatment plans.
- Benefits:
- Enhanced patient care through tailored treatment plans.
- Predictive analytics for early disease detection.
- Streamlined operations, reducing wait times and costs.
Such applications ensure not only better health outcomes but also cost savings for both healthcare providers and patients. However, the reliance on big data also brings challenges, particularly concerning the security and privacy of sensitive medical information.
Financial Services
Financial institutions, on the other hand, use big data tools to enhance decision-making processes and manage risks effectively. With tools like Tableau and various machine learning algorithms, banks and fintech companies can analyze customer transactions in real time. This not only helps in detecting fraud quickly but also assists in identifying trends that inform product offerings.
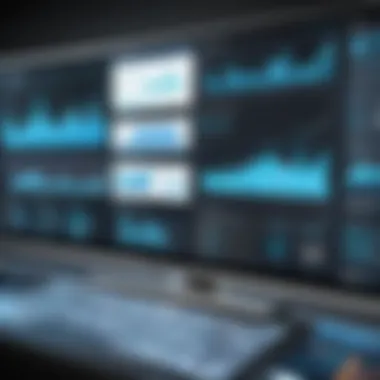
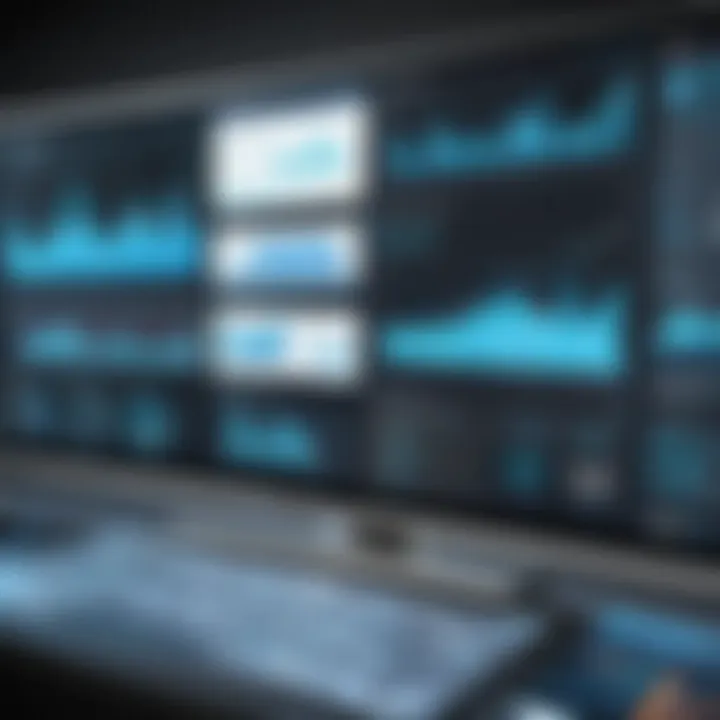
- Considerations:
- Data integrity is paramount to avoid misinformed decisions.
- Compliance with regulations like GDPR is critical.
For instance, when a potential fraud is detected, systems using Apache Hadoop can rapidly analyze past transactions to confirm the anomaly. This leads to rapid preventive measures, safeguarding customer assets and trust in financial services.
Retail Sector
The retail industry is another sphere where big data analysis tools shine. Businesses utilize tools for analyzing purchasing patterns, inventory management, and customer behavior. For instance, retailers leverage ETL tools to gather data from various sources, transforming it into actionable insights.
- Applications:
- Improved customer engagement through personalized marketing.
- Forecasting inventory needs to minimize stockouts and overstock.
- Optimizing supply chain logistics based on real-time data.
A notable example might be the way large chains, like Walmart, utilize big data analytics to decide how many units of a product to carry based on local shopping trends. By understanding what sells and what doesn’t, they optimize their inventory management, ultimately enhancing customer satisfaction and loyalty.
In summation, the real-world applications of big data tools are as diverse as they are critical. With each sector facing unique challenges and opportunities, the ability to analyze and derive value from data is more important than ever. As technology continues to evolve, so too will the methods and tools that industries use to harness big data effectively.
Challenges in Big Data Analysis
In the ever-evolving landscape of data analytics, challenges often lurk around every corner. Understanding these challenges is crucial, as they can greatly determine the success or failure of data projects. When grappling with big data, organizations face both technical and non-technical hurdles that need addressing. Ignoring these might lead to ineffective analysis and subpar results, which can be detrimental in today’s data-driven world.
Data Quality Issues
Data quality is the backbone of any analytical endeavor. No matter how robust your tools or analytics methods, if the data isn't reliable, it’s like trying to build a house on quicksand. Poor data quality can arise from various sources—errors during data entry, outdated information, or inconsistency across datasets. These can lead to skewed insights, causing strategic missteps.
Moreover, ensuring data quality often requires a steady allocation of time and resources. Out of the gate, organizations might underestimate the significance of data cleaning and preparation. Many data analysts often spend a considerable chunk of their time on these preliminary steps rather than on analysis itself. This can introduce delays in project timelines, increase costs, and lead to frustration throughout the team.
It's worth noting that a study on data-related failures indicated that around 60% of data projects don't meet business objectives primarily due to poor data quality. Hence, employing automated data cleaning tools and validating data through consistent checks is not just beneficial; it’s essential.
Some common strategies to address data quality issues include:
- Establishing Clear Standards: Develop guidelines on data entry and usage across the organization.
- Regular Audits: Schedule periodic reviews of data to identify and correct inconsistencies.
- Data Governance: Implementing measures that determine data ownership and accountability can help keep the data in check and relevant.
Skill Gaps
As organizations lean more heavily into data analytics, one of the significant challenges they encounter is the skill gap within their teams. While the tools themselves may be sophisticated, they still require a workforce that's well-versed in using them. Many professionals may have foundational knowledge but lack the specific expertise needed for certain big data tools or methodologies. This disconnect can lead to underutilization of resources, wasted investments, and ultimately, lost opportunities.
Additionally, with technology advancing at a breakneck pace, skills taught just a few years ago may no longer suffice. This ever-changing arena necessitates continuous learning and adaptation. Businesses may find themselves scrambling to keep their staff trained, often resulting in hiring freezes or heavy reliance on external consultants or training programs.
To mitigate skill gaps, organizations might consider:
- Upskilling Existing Staff: Offering training sessions, access to online courses, or workshops can help elevate the skill level of current employees.
- Recruiting Specialized Talent: Actively seeking out individuals with specific expertise can help balance the overall skill set of the team.
- Encouraging a Culture of Continuous Learning: Creating an environment that promotes growth and development can engender more innovative approaches to tackling data challenges.
Closing Thought: In today’s intricate world of big data, addressing both data quality issues and skill gaps is not merely a best practice but a vital step towards achieving effective data analysis that drives informed business decisions.
Future Trends in Big Data Tools
The realm of big data is ever-evolving, and staying updated with the latest trends becomes crucial for businesses looking to stay competitive. As technology continues to advance, the tools and methodologies used for big data analysis are also shifting. Recognizing these trends allows companies, whether they're fledgling startups or established enterprises, to make informed decisions about the tools they adopt. Here, the focus is on two significant trends: AI and automation, as well as an increased emphasis on data ethics.
AI and Automation
With the rise of artificial intelligence, big data tools are rapidly incorporating automation features. By leveraging AI, these tools can analyze vast datasets more efficiently than humans ever could. Automation not only accelerates data processing but also enhances accuracy by minimizing human error. For instance, companies can utilize machine learning algorithms to automate repetitive tasks such as data cleansing and feature extraction. This frees up time for data scientists and analysts, allowing them to concentrate on high-level strategic initiatives.
Furthermore, AI enables predictive analytics, which can offer businesses foresight into trends and behaviors. For example, retailers can analyze customer purchasing patterns to predict future sales and stock requirements. This is invaluable for optimizing inventory and ensuring that businesses stay ahead of demand.
As these technologies evolve, they promise not just to streamline operations but also to provide granular insights that inform decision-making at all levels. The automation of big data tasks can lead to significant cost savings while ensuring that insights are derived in real-time, thus making organizations more agile.
Increased Focus on Data Ethics
The surge in data collection has led to heightened concerns about privacy and the ethical use of data. In the past, many companies operated without clear ethical guidelines, leading to significant issues regarding consumer trust. As consumers become more aware of how their data is used, organizations are now compelled to adopt more responsible data practices.
This trend is manifesting itself in various ways:
- Transparency: Firms are increasingly expected to provide clear information on how data is collected, used, and stored. This builds trust and fosters better relationships with consumers.
- Regulatory Compliance: The implementation of regulations like the General Data Protection Regulation (GDPR) has forced organizations to rethink their approach to data management. Compliance is no longer just about avoiding penalties; it’s about ethical accountability.
- Data Stewardship: Organizations are beginning to view data as an asset that requires careful management. This includes not just securing data but also ensuring that its usage aligns with ethical standards.
"Ethics in data analysis is not just a legal requirement but a pivotal part of building long-lasting consumer relationships."
By focusing on data ethics, companies can not only avoid pitfalls but also establish themsleves as industry leaders committed to responsible practices. The emphasis on ethics is likely to grow, changing the landscape of big data analysis profoundly.
Finale
In this fast-paced world where data is king, the tools available for analyzing big data have become crucial for businesses looking to stay ahead of the curve. The insights derived from robust data analysis tools not only help organizations make informed decisions but also unlock new avenues for growth and innovation. This article has brought to light various aspects of big data tools, showcasing how crucial it is for professionals to adapt and evolve.
Recap of Key Points
- Understanding Big Data: It is essential to grasp what constitutes big data and why analyzing it holds paramount importance in various industries. The sheer volume, velocity, and variety it encompasses can be overwhelming.
- Diverse Tools Available: Tools like Apache Hadoop and Tableau cater to different analytical needs, from statistical analysis to data visualization. Choosing the right tool depends on specific business goals and the nature of the data involved.
- Factors to Consider: Elements such as scalability, integration with existing systems, and user-friendliness are crucial. Furthermore, businesses should also assess technical capabilities against budget limitations.
- Challenges Ahead: Companies often grapple with data quality and the skill gap within their teams. Addressing these issues effectively can significantly improve the overall analysis results.
These points illustrate that selecting an appropriate tool is not just about the features it provides but understanding its fit within the broader context of business needs and limitations.
Final Thoughts on the Future of Big Data Analysis
Looking ahead, the landscape of big data analysis will continue to transform, driven by advancements in AI and automation. As these technologies become more sophisticated, they will enable businesses to derive insights faster and more accurately than before.
Moreover, as companies increasingly focus on ethical data practices, there will be a stronger emphasis on data governance. The ability to maintain compliance and ensure data integrity will become indispensable for organizations.