Predictive Analytics Visualization: Unlocking Insights
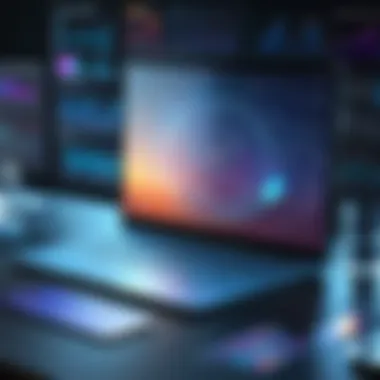
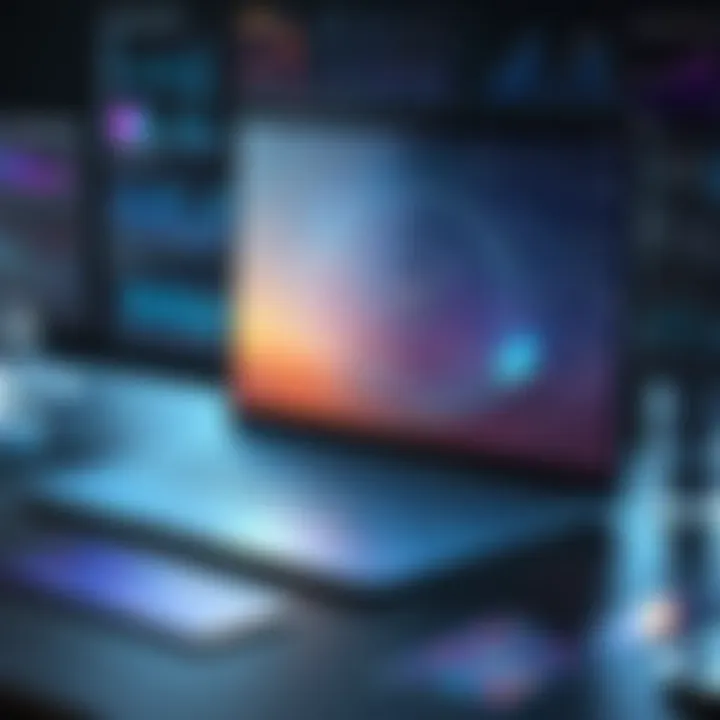
Intro
Predictive analytics visualization sits at the intersection of data science and business intelligence. It involves using advanced analytical techniques to predict future outcomes based on historical data, culminating in visual representations that foster better understanding and decision making. In a world overwhelmed by data, mastering visualization becomes essential for both business professionals and IT experts.
This article will unpack the methodologies that underline predictive analytics and the visualization techniques that enhance comprehension. As organizations increasingly rely on data for strategic decisions, effective visualization not only clarifies complex data but also compels action.
By the end of this discussion, readers will gain insight into the frameworks and best practices for leveraging predictive analytics visualization, ultimately equipping them with the knowledge needed to harness the full power of their data.
Understanding Predictive Analytics
Predictive analytics is a cornerstone of modern data-driven decision-making. Its ability to forecast future events based on historical data makes it invaluable across various industries. In this section, we will examine the definition, historical context, and essential components that frame predictive analytics.
Definition and Scope
Predictive analytics is a branch of advanced analytics that focuses on predicting future outcomes based on historical data and statistical algorithms. It combines traditional data analysis with machine learning models to identify patterns and trends. This scope excludes mere descriptive statistics, emphasizing understanding the causes behind future trends. The core benefit of predictive analytics is its ability to inform strategic decisions, ultimately leading to improved business performance.
Historical Context
The journey of predictive analytics has evolved significantly over the decades. It began in the early 20th century with basic statistical methods used in fields like finance and healthcare. The introduction of computers in the mid-20th century revolutionized the field, allowing for complex calculations that could manage extensive datasets. As technology progressed, the integration of machine learning in the late 20th century propelled predictive analytics forward. This historical backdrop is crucial, as it provides context for understanding how predictive analytics has become more sophisticated and accessible today.
Key Components
Statistical Techniques
Statistical techniques lay the groundwork for predictive analytics. They involve methods such as regression analysis, time-series analysis, and hypothesis testing. A key characteristic is their ability to handle and interpret data. These techniques are beneficial due to their long-standing applications in various industries. They provide a strong mathematical basis, allowing for accuracy in predictions. However, they may require a significant level of expertise, which can be a barrier for novice users.
Machine Learning Algorithms
Machine learning algorithms represent a pivotal aspect of predictive analytics. They adapt through experience, enhancing their predictive power as they process more data. These algorithms include decision trees, neural networks, and support vector machines. Their flexibility and scalability make them a popular choice for modern data applications. Moreover, they can uncover complex patterns traditional methods might miss. However, they also pose challenges, such as the need for substantial computational resources and the risk of overfitting the model to the training data.
Data Mining Methods
Data mining methods focus on discovering patterns in large datasets. Techniques like clustering, association rule learning, and anomaly detection fall under this umbrella. A defining feature of data mining is its capability to handle unstructured data, identifying insights that can inform predictive models. Its widespread use in sectors like retail and telecommunications highlights its effectiveness. Yet, it can also lead to issues such as false positives, complicating the analysis process.
"The integration of statistical techniques, machine learning algorithms, and data mining methods together forms a robust framework for effective predictive analytics."
Fundamentals of Data Visualization
Data visualization stands as a pillar within predictive analytics. Its significance cannot be overstated when it comes to interpreting complex data sets. Effective visualization helps in unraveling patterns, trends, and outliers that raw data might obscure. Furthermore, it plays a vital role in translating intricate numerical findings into understandable visuals that can be utilized for informed decision-making.
Visualizing data helps convert data overload into clarity. As the volume of data expands, the ability to quickly discern insights becomes essential. Good visual presentation can mitigate the risk of misinterpretation while enhancing the viewer's understanding. Organizations must adapt these practices to remain competitive in today's data-driven landscape.
Importance of Visualization in Data Analysis
The visualization of data is critical in the analysis process. It aids users in grasping complex ideas intuitively. For instance, data represented through visual format allows analysts to identify correlations or discrepancies swiftly. Without such tools, valuable information may remain hidden within vast datasets.
Moreover, visualization fosters communication. Whether sharing findings with stakeholders or presenting to a broader audience, visuals facilitate better discussions. They act as a common language, bridging gaps between technical and non-technical team members.
Types of Data Visualizations
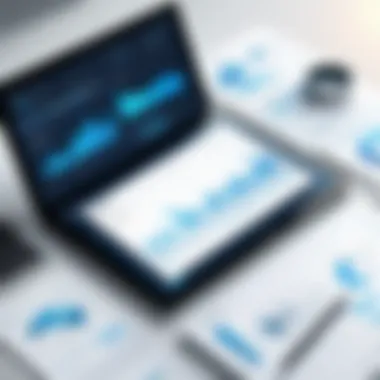
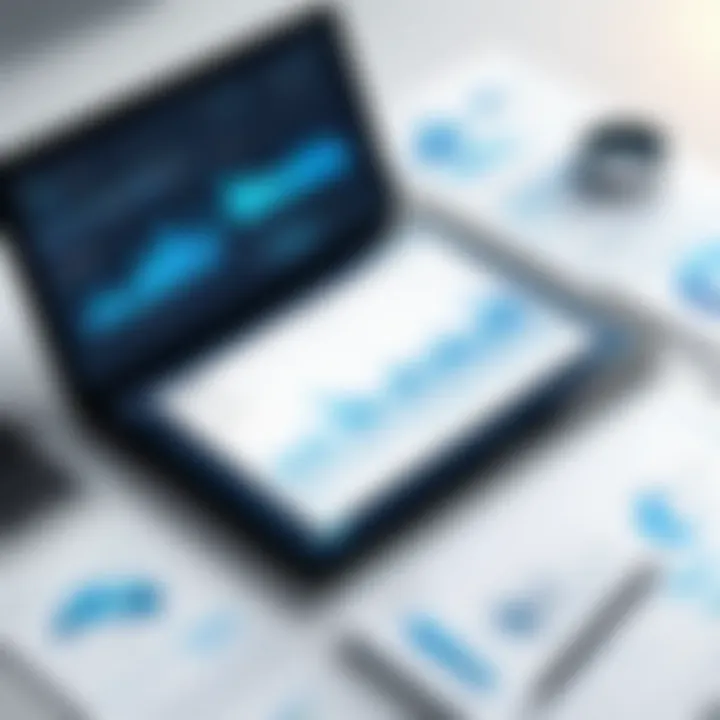
Data visualizations come in various forms, each serving unique purposes. Understanding these options is crucial for effective presentation.
Charts and Graphs
Charts and graphs are indispensable tools in data visualization. They are effective in summarizing large data sets into digestible formats. A key characteristic of these methods is their ability to simplify complex relationships. For predictive analytics, they allow analysts to visualize trends over time and compare discrete data points effortlessly.
The unique feature of charts and graphs lies in their versatility. They can be used for both qualitative and quantitative data, making them suitable for a variety of applications. One advantage is their relatively straightforward design process. However, over-reliance on charts can lead to confusion if not chosen correctly. Hence, clarity in design is paramount.
Heat Maps
Heat maps provide another valuable visualization method. They display data intensity through color variations. A primary characteristic of heat maps is their ability to convey volumes of data at a glance. This is particularly beneficial when integrating numerous variables.
The unique feature of heat maps is their spatial representation of data. They excel at revealing patterns that might not be observable in 'traditional' graphs. However, an area to be mindful of is their potential for misinterpretation. If viewers lack context about color ranges, the visual representations may mislead them.
Dashboards
Dashboards offer a comprehensive view into data analysis. They aggregate various visuals into one interactive interface, providing an overview of key metrics. This is crucial for real-time decision-making within organizations.
Dashboards' defining feature is their interactive nature. They allow end-users to delve into specific elements when necessary, providing both high-level summaries and detailed insights. The primary advantage of dashboards is user engagement and the proactive approach they promote. Yet, the challenge lies in their design complexity. Poorly designed dashboards can overwhelm users with information, detracting from their intended purpose.
Best Practices for Effective Visualization
Creating impactful visualizations entails adhering to several best practices. Firstly, simplicity is key. Uncluttered designs enhance readability. Secondly, ensure that color choices are deliberate and consistent across visual materials.
Additionally, interactivity can deepen engagement. Allow user manipulation to explore data facets on their own terms. Finally, always provide context, as it transforms data from symbols into meaning.
"The aim of visualization is not just to show data but to make it clear and actionable."
Best practices are fundamental for successful data visualization. They elevate the quality of insights drawn from the predictive analytics process.
The Intersection of Predictive Analytics and Visualization
Predictive analytics relies on a wealth of data and advanced algorithms to forecast future outcomes. However, the mere presence of sophisticated analytics does not guarantee understanding or insight. This is where visualization plays a crucial role. Visual representation of data can significantly enhance comprehension by transforming complex datasets into intuitive formats.
Visualization enables users to see patterns, trends, and anomalies that may go unnoticed in raw data. This is particularly important for decision-makers who may not have technical backgrounds. By providing a clearer view of predictive models, visualization facilitates more informed decision-making. In this section, we will explore how various visualization techniques empower predictive analytics and improve business practices.
How Visualization Enhances Predictive Models
Visualization enhances predictive models by making complex data relationships more accessible. When applied correctly, it clarifies the results derived from statistical analyses. For instance, linkage diagrams and scatter plots can visualize correlations, allowing stakeholders to grasp key insights quickly.
Moreover, effective visualization can help in troubleshooting predictive models. If a model is underperforming, visual methods can assist data scientists in pinpointing where issues lieβbe it in data quality, model complexity, or feature selection. This feedback loop is vital for refining predictive analytics, underscoring the reciprocal relationship between analytics and its visualization.
Tools for Predictive Analytics Visualization
In the realm of predictive analytics, visualization tools are essential for transforming complex data sets into comprehensible insights. Choosing the right visualization tool can enhance data interpretation and facilitate better decision-making for businesses and organizations. The effectiveness of predictive models largely depends on how the data and outcomes are presented. This section will explore the leading software solutions available in the market today and will guide users in selecting the most suitable tool for their specific needs.
Popular Software Solutions
Tableau
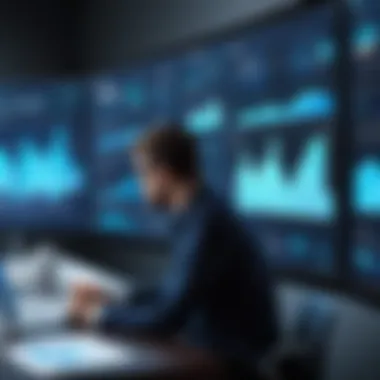
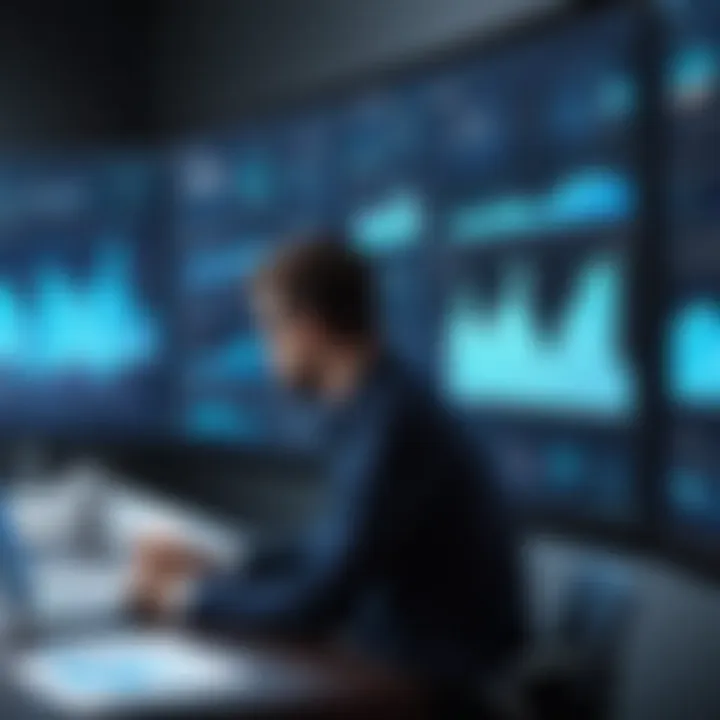
Tableau is a powerful data visualization tool known for its user-friendly interface and diverse visualization options. It allows users to create interactive and shareable dashboards. The key characteristic of Tableau is its capability to handle large sets of data efficiently. Users can drag and drop fields to create stunning visualizations without needing extensive programming skills. A significant advantage of Tableau is its ability to connect with various data sources like Excel, SQL databases, and cloud services. However, it may require substantial investment compared to other options. While Tableau offers many unique features, its high licensing cost can be a barrier for smaller organizations.
Microsoft Power BI
Microsoft Power BI stands out for its seamless integration with other Microsoft products. This aspect makes it increasingly beneficial for organizations already using tools like Excel or Azure. Its standout characteristic is the ability to share insights easily through the Microsoft ecosystem. Users appreciate the intuitive design, which allows for quick report generation. A unique feature of Power BI is the Natural Language Query (NLQ) capability that enables users to ask questions in plain language, thereby simplifying data retrieval. One disadvantage is that advanced analytics features may not be as robust as those in other tools.
R and Python Libraries
R and Python libraries, such as ggplot2 for R and Matplotlib and Seaborn for Python, provide powerful options for those with programming expertise. These libraries allow for customizable visualizations tailored to specific analytical needs. A key characteristic is the flexibility they offer in creating virtually any type of visual representation. Users can manipulate data deeply and apply complex statistical methods. However, the downside is that they require a solid understanding of programming, which can deter non-technical users or beginners. Despite this, R and Python libraries are popular among data scientists for their versatility and depth.
Selecting the Right Tool
Choosing the right visualization tool can be daunting, as there are many factors to consider. Key considerations include ease of use, cost, and specific visualization capabilities. It's important to assess the technical skill level of the users who will be working with the tool. For instance, organizations with non-technical users might prefer Tableau or Microsoft Power BI for their user-friendly design. Conversely, teams with strong programming backgrounds might lean toward R or Python for greater customization.
Furthermore, evaluating the specific data sources to be used is crucial. Some tools integrate seamlessly with existing databases, while others may require extra steps for data manipulation.
"Effective visualization is pivotal for harnessing the full potential of predictive analytics."
The choice of visualization tool should align with the organizational goals, available resources, and user capabilities. Understanding each tool's strengths and weaknesses will ultimately lead to more informed decisions and better analytical outcomes.
Challenges in Predictive Analytics Visualization
Predictive analytics visualization possesses the potential to revolutionize decision-making processes, but it also comes with its own set of challenges. Recognizing and addressing these challenges is essential for achieving optimal results. This section will explore some critical issues, such as data overload, misinterpretation of data, and technical barriers that can hinder effective visualization. Understanding these challenges can enhance decision-making and improve the accuracy of valuable insights derived from data.
Data Overload
Data overload arises when users are inundated with excessive information from various sources, rendering it difficult to draw meaningful conclusions. In predictive analytics visualization, this issue is especially prevalent due to the sheer volume of data being collected and analyzed. Decision-makers may find themselves facing intricate dashboards cluttered with insufficiently curated visuals that hide essential trends and patterns.
- Implications of Data Overload: The cognitive load on users increases, making it harder to focus on what is relevant. Consequently, critical insights may become obscured amidst the confusion.
- Strategies to Combat Overload: Employing filtering techniques is vital. Prioritizing key indicators allows users to concentrate on significant data without feeling overwhelmed. Simplifying visuals and maintaining a clear layout also facilitate better comprehension. On-demand access to detailed information can help keep dashboards concise while still allowing deeper exploration when needed.
Misinterpretation of Data
Misinterpretation of data presents a critical challenge in predictive analytics visualization. As users engage with complex visuals, there is a risk of drawing incorrect conclusions based on misleading representations. Different users may hold disparate interpretations due to varied experiences and expertise.
- Contributing Factors: Ambiguous visuals, such as poorly labeled axes or convoluted graph types, can lead to confusion. Additionally, confirmation bias may cause users to seek only the information that supports their preconceived notions, distorting interpretations further.
- Preventive Measures: Clear and effective communication of data is paramount. Training users on best practices in data interpretation can reduce the likelihood of errors. Consistent formats and intuitive design choices can aid comprehension and foster an accurate understanding of analytics.
"The challenge is not the data itself, but how we visualize it for effective decision-making."
Technical Barriers
Technical barriers often serve as impediments to effective predictive analytics visualization. These could stem from a lack of proficiency in utilizing visualization tools or inadequate infrastructure to support advanced analytical processes. Some organizations may also encounter challenges regarding integrating new visualization software with existing systems.
- Consequences of Technical Barriers: When users struggle with technology, the analytical capabilities can be squandered. Even the most sophisticated models may yield little benefit if users cannot effectively translate insights into actionable strategies.
- Solutions: Providing comprehensive training on the tools ensures users can make the most of the available resources. Furthermore, investing in compatible tech infrastructures allows for seamless integration, fostering a smooth experience when transitioning to advanced visualization platforms.
Addressing these challenges in predictive analytics visualization is necessary for effective decision-making. Navigating data overload, minimizing misinterpretation, and overcoming technical barriers can help organizations leverage data insights to drive success.
The Future of Predictive Analytics Visualization
Predictive analytics visualization is poised for significant evolution. The way data is interpreted and represented will become more advanced. Increasing demands for real-time insights drive businesses to adopt innovative strategies. Visualization tools are not only about displaying data but also about enhancing decision-making processes. Companies need to harness the power of visual analytics to ensure they stay ahead in competitive industries.
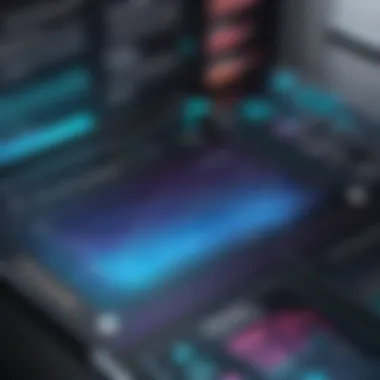
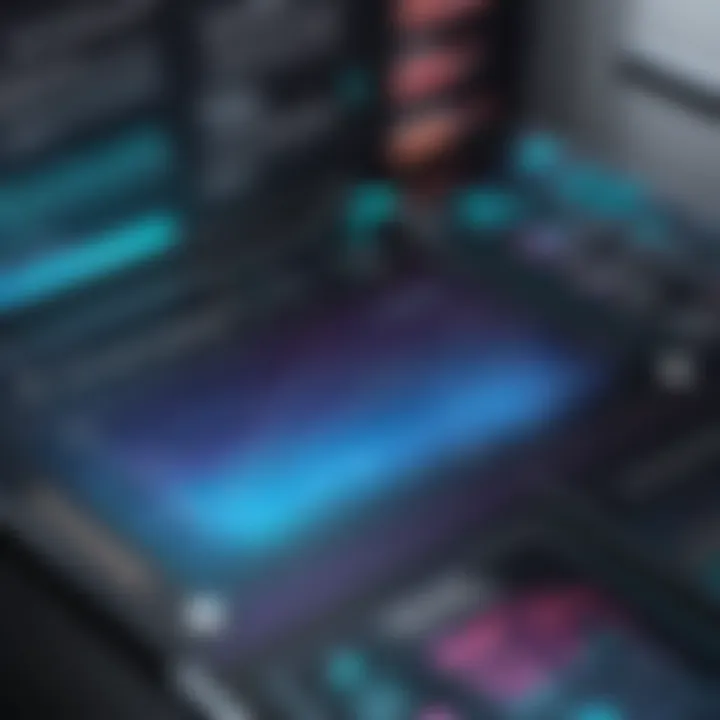
Emerging Trends
Understanding emerging trends is essential for anticipating future developments. One noticeable trend is the incorporation of interactive visualizations. These tools allow users to engage with data directly and manipulate it according to their needs. This leads to better understanding and immediate insights.
There is also a growth in the use of augmented analytics. By leveraging automation and machine learning, this approach enhances the analytics process. Users can gain insights quickly, as the system identifies patterns without extensive manual input.
More organizations are prioritizing real-time data visualization. The ability to access and analyze data instantaneously streamlines decision-making. This trend supports businesses in adapting swiftly to changes in the market environment, enhancing their adaptive capabilities.
Integration with Other Technologies
Integrating predictive analytics visualization with various technologies strengthens its effects. Different technological advancements bring unique advantages.
Artificial Intelligence
Artificial intelligence is a vital player in the future of predictive analytics visualization. It offers automation in data analysis. This ability to process large datasets efficiently enhances decision-making capabilities. AI enables predictive models to continually learn and improve from new data inputs. This quality makes AI a compelling choice in reflective analytics.
However, reliance on AI can also present challenges. Misinterpretation of output generated by AI models is a concern. Proper understanding is essential to maximize benefits. Users must develop skills to discern AI-generated insights effectively.
Internet of Things
The Internet of Things (IoT) plays an integral role in enriching predictive analytics visualization. With numerous devices generating data effortlessly, IoT enables deeper data insights. As more devices connect to the internet, the amount of usable data increases. This vast amount of data aids in more accurate predictive analyses.
Nonetheless, IoT data can be overwhelming. Managing and analyzing this information requires robust strategies to avoid analysis paralysis. Therefore, organizations must ensure they have the right tools and frameworks in place to harness IoT effectively.
Big Data
Big Data significantly influences predictive analytics visualization. The ability to analyze vast volumes of data is essential for accurate insights. Big Data allows for a more comprehensive view of trends hitting various industries. This helps businesses in recognizing key opportunities and threats.
The challenge, however, lies in data quality. Poor-quality data can lead to inaccurate predictions. Framing effective data governance policies becomes crucial for maximizing the strengths of Big Data within predictive analytics.
By understanding these technologies and trends, organizations can capitalize on the future of predictive analytics visualization. This forward-thinking approach will support more informed, rapid decision-making in changing environments.
End
The conclusion acts as a crucial component in encapsulating the essence of predictive analytics visualization. It is not merely an endpoint; it serves as a summary of the key insights gained throughout the article. This synthesis is vital for reinforcing understanding and ensuring that the reader can carry forward relevant concepts into practical applications.
In this context, several specific elements are pivotal. Firstly, recapping the significance of predictive analytics visualization illustrates how it facilitates data-driven decision-making. It highlights that effective representation of data enhances comprehension and enables stakeholders to derive actionable insights efficiently.
Secondly, addressing the methodologies and tools discussed earlier emphasizes the importance of selecting the right solutions in optimizing analytical efforts. Understanding the variety of tools such as Tableau and Microsoft Power BI can empower businesses to make informed choices that align with their objectives.
Finally, the considerations surrounding future trends in predictive analytics visualization, particularly those related to the integration with emerging technologies, underline the dynamic nature of this field. Organizations must remain adaptable.
"In a world awash in data, the ability to visualize that data effectively becomes not just an advantage, but a necessity."
Thus, the conclusion solidifies the overarching narrative of the article, paving the way for practical application and indicating the imperative for continuous engagement with evolving methodologies.
Summation of Key Points
- Predictive analytics visualization is crucial for data-driven decision-making.
- Effective tools are available, like Tableau and Microsoft Power BI, which enhance predictive models.
- Understanding emerging trends is essential for adapting to new technologies.
Call to Action for Businesses
Businesses ought to leverage predictive analytics visualization more strategically. This involves:
- Investing in Training: Encouraging staff to develop skills in utilizing tools effectively can increase overall efficiency.
- Adopting Best Practices: Aligning visualization practices with organizational goals ensures that insights are both relevant and actionable.
- Staying Informed: Monitoring trends in predictive analytics and integrating new technologies can present substantial opportunities for growth.