Understanding Data Lake Pricing: Costs and Considerations
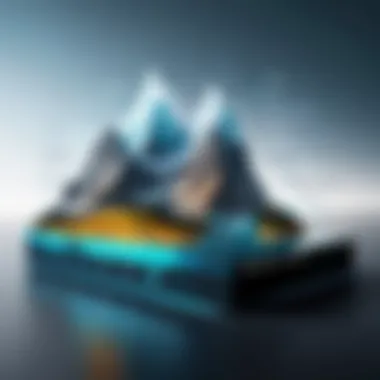
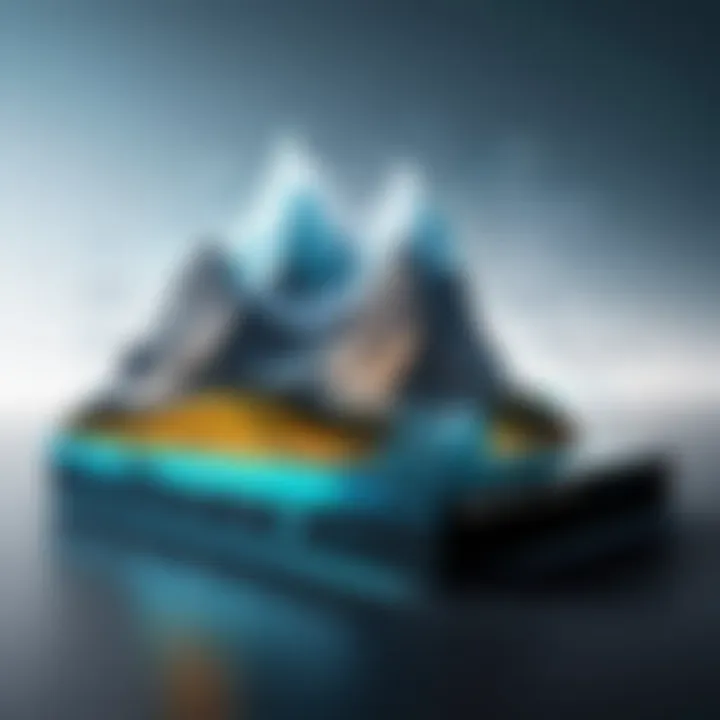
Intro
Data lakes have emerged as vital components in the landscape of data management. Their ability to store vast amounts of structured and unstructured data is attractive for businesses aiming to leverage analytics for decision-making and competitive advantage. However, navigating the complexities of data lake pricing can be challenging. Understanding costs associated with these digital repositories requires a dissecting of various elements that influence overall expenses. This article ventures into the key considerations and costs related to data lake pricing, aiming to equip decision-makers with critical insights.
Overview of Key Features
When evaluating data lake options, several key features play a fundamental role. Understanding these features allows organizations to select the most suitable data lake that aligns with their needs and budget.
Essential Software Capabilities
A proficient data lake must offer essential capabilities. These include:
- Scalability: The ability to adjust storage and processing power as data needs grow.
- Data Ingestion: Efficient handling of diverse data formats from various sources, including IoT devices, databases, and web applications.
- Data Governance: Tools that ensure compliance, security, and data quality management.
- Analytics Integration: Ability to work seamlessly with analytical tools for data exploration and reporting.
These capabilities are essential as they dictate how well a data lake can serve an organization’s evolving requirements.
Unique Features that Differentiate Options
Different service providers may offer unique features that can be pivotal in decision-making. Some options to consider include:
- Multi-Cloud Support: Some platforms provide flexibility to operate across multiple cloud environments, reducing vendor lock-in.
- Machine Learning Enhancements: Features that facilitate the integration of machine learning capabilities can enhance data analysis.
- Real-Time Processing: Options for real-time data ingestion and processing can be crucial for businesses that need timely insights.
Businesses should evaluate these features against their specific needs to determine which solutions can drive the most value.
User Experience
User experience plays a significant role in the effectiveness of a data lake solution. A favorable experience often stems from intuitive design and robust support resources.
Interface and Usability
The interface of a data lake platform impacts users’ ability to adopt and utilize the system. A clear and engaging interface promotes ease of use, while complex interfaces can hinder adoption. Key factors include:
- Navigation: Straightforward menus and logical workflows allow for easy exploration of the platform’s capabilities.
- Visualization Tools: Effective data visualization options aid in interpreting data effectively.
A well-designed user interface reduces the learning curve and enhances productivity.
Support and Community Resources
Having access to support and community resources is crucial. Options include:
- Documentation: Comprehensive guides and documentation help troubleshoot and optimize utilization.
- Community Forums: Platforms like Reddit and specialized forums can provide valuable insights and peer support.
A strong support system enhances user experience and can greatly impact overall satisfaction with the data lake solution.
Understanding both functionality and user experience can significantly impact the decisions organizations make regarding data lakes.
As discussions around data lake pricing deepen, it becomes clearer that multiple factors influence costs. By dissecting essential capabilities, unique features, and user experience, organizations can start to navigate the complexities of data lake pricing in a more informed way.
Understanding Data Lakes and Their Importance
Data lakes have transformed the way organizations manage and analyze vast amounts of data. Through their ability to store structured and unstructured data in a single, scalable location, they have become a backbone for modern data strategies. Understanding data lakes is crucial for decision-makers and IT professionals alike, as they navigate the nuances of data management and pricing.
One of the primary benefits of data lakes is their flexibility. Unlike traditional data storage systems, data lakes allow organizations to ingest data from various sources without the need for upfront structuring. This characteristic enables them to accommodate data in its original form, which is vital for analytics, machine learning, and business intelligence. Moreover, data lakes support a range of data types, including social media content, IoT sensor data, and log files, making them invaluable for businesses aiming to leverage big data.
However, understanding the structure and implications of data lakes is not merely technical jargon. It involves recognizing the importance of data governance and quality. Without overseeing data in a data lake, organizations can face challenges with data integrity and compliance, especially as regulations around data usage become stricter. Proper governance ensures that the data is both secure and usable, enhancing the overall value extracted from these repositories.
Thus, an in-depth understanding of data lakes allows organizations to position themselves better within the competitive landscape. It empowers them to formulate strategic decisions regarding their data architecture and budgeting. As they realize the significance of data lakes in modern data management, they become more equipped to harness the full potential of their data assets.
Definition of a Data Lake
A data lake can be defined as a centralized repository that allows for the storage of vast amounts of raw data in its native format until needed. Unlike data warehouses, which store data in a structured manner, data lakes provide an environment for both structured and unstructured data. This design facilitates easy access and analysis without the need for conversion or transformation beforehand.
The architecture of a data lake often leverages cloud storage solutions, which can scale according to demand. This scalability is a considerable advantage, allowing organizations to accommodate growing data volumes without the limitations of hardware constraints.
The Role of Data Lakes in Modern Data Management
The role of data lakes in modern data management is integral and multi-faceted. By enabling organizations to store and analyze massive amounts of diverse data, data lakes serve as the foundation for advanced analytics and decision-making processes.
Data lakes support a wide range of applications, from complex querying and reporting to machine learning and real-time analytics. For instance, an e-commerce company could analyze user behavior from multiple sources, such as website logs and social media interactions, to improve marketing strategies and enhance customer experiences.
Moreover, data lakes foster collaboration among different departments by providing a shared platform for analytics. Teams across sales, marketing, and customer support can access the same data while using it to drive specific initiatives relevant to their roles.
Overview of Data Lake Pricing Models
In the evolving landscape of data management, understanding pricing models for data lakes is crucial for organizations looking to optimize their data strategies. Different pricing models can significantly impact the overall cost, making it essential for decision-makers to evaluate the nuances of each. The right model not only affects expenditures but also drives operational efficiency. This overview will present the primary models: consumption-based, subscription-based, and tiered pricing structures. Each of these models offers unique benefits and considerations that can align with varying business needs.
Consumption-Based Pricing
Consumption-based pricing allows organizations to pay based on the storage and services they actually use. This model is appealing for companies with fluctuating data needs. For instance, businesses expecting variable workloads can benefit from an approach that scales with demand. With this model, there is less risk of overpaying for resources that remain unused.

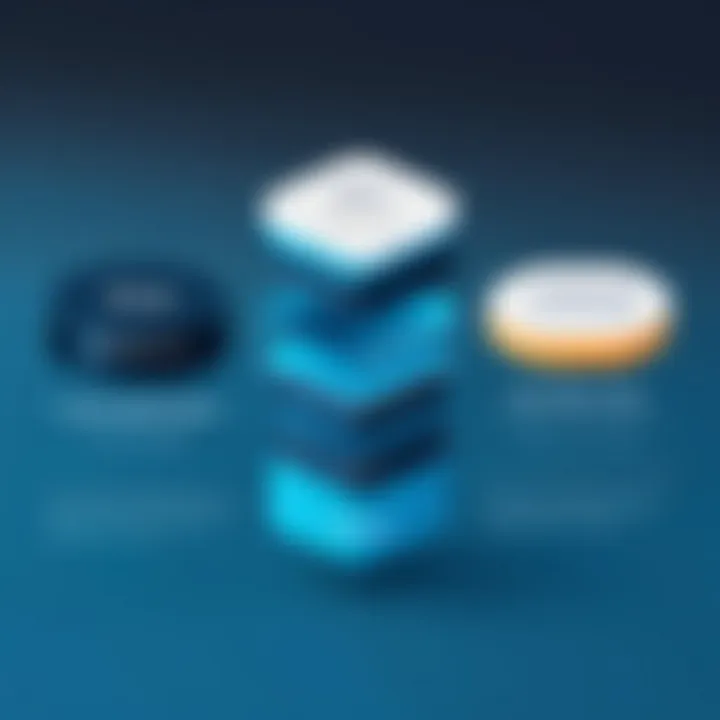
This pricing can involve several cost components, including:
- Storage costs based on the total volume of data stored.
- Data transfer fees for moving data in and out of the lake.
- Costs related to processing data or querying usage.
One major advantage of this structure is flexibility, allowing businesses to manage costs effectively during low-usage periods. However, it can lead to unpredictable expenses if data consumption spikes unexpectedly. This model works well for organizations willing to monitor their usage closely and adjust their strategies accordingly.
Subscription-Based Pricing
Subscription-based pricing is a fixed-rate approach where organizations pay a set fee for access to a data lake service over a specific period. This model provides predictability in budgeting, which can be highly beneficial for organizations that need reliable cost forecasting. Typically, these subscriptions may include a set amount of storage and certain quotas for data transfer and processing.
Advantages of subscription-based pricing include:
- Predictable budgeting: Organizations can plan their finances without surprise costs.
- Simplified billing: By providing a fixed rate, it minimizes complexity in understanding the various charges.
However, a subscription model might not be as beneficial for companies with fluctuating usage patterns. They may end up paying for excess capacity. Therefore, businesses need to carefully evaluate their data requirements before committing to a subscription pricing model.
Tiered Pricing Structures
Tiered pricing structures offer a hybrid approach, combining elements of both consumption and subscription-based pricing. In this model, organizations pay for different levels of service or data usage, with costs decreasing per unit as usage increases. This is particularly attractive for enterprises that anticipate substantial growth in data volume over time.
Some characteristics of tiered pricing include:
- Different payment rates depending on usage thresholds, incentivizing increased data usage.
- Enhanced scalability, as organizations can select plans that align with their projected growth.
While tiered pricing can effectively balance costs among varying usage levels, organizations must be vigilant about monitoring their data to ensure they do not surpass usage tiers unintentionally. Understanding the specific structures offered by different providers is crucial for making informed decisions that align with business goals.
"Choosing the right pricing model can significantly influence the overall success of your data lake initiative. It’s essential to align cost structures with actual data requirements."
Factors Influencing Data Lake Costs
Understanding the various factors that influence data lake costs is crucial for organizations looking to implement or manage these complex systems. Each element contributes to the overall financial commitment, impacting both short-term and long-term budgeting. By grasping these factors, businesses can make informed decisions on their data strategies, optimizing usage and reducing unnecessary fees.
Storage Costs
Storage is one of the primary considerations when assessing data lake costs. The amount of data generated and stored directly correlates to the expenses incurred. Providers like Amazon Web Services, Microsoft Azure, and Google Cloud Platform offer different types of storage solutions, ranging from simple object storage to more advanced data warehousing functionalities. The pricing for these services often varies based on the scalability options and redundancy requirements chosen.
For instance, the cost can be influenced by what kind of storage service you use. Some options may be cheaper but may lack the performance needed for large-scale analytics operations. On the other hand, premium storage solutions promise higher performance but come with higher costs. Therefore, it is essential to analyze both current and forecasted storage needs to choose a suitable option without overspending.
Data Transfer Fees
Data transfer fees can quickly accumulate, adding another layer to the cost structure associated with data lakes. Charges often apply when transferring data out of the cloud services toward other entities or regions. This is something many business professionals overlook until they face unexpected bills. Moving large datasets frequently incurs additional costs; hence, understanding the volume of data being transferred is pivotal for cost management.
Companies must consider both ingress (data entering) and egress (data exiting) as they will impact the total expenditure. Some providers offer free ingress but may charge for egress, necessitating careful planning of data flow to minimize costs.
Access and Retrieval Costs
Accessing and retrieving data from a data lake can also entail costs that vary based on the frequency and methods of access. Providers often have different pricing tiers for how quickly and efficiently data can be retrieved. For example, frequent access to hot data may cost significantly more than accessing cold data, which is stored in lower-cost, slower tiers.
It is advisable to categorize data based on access patterns. Identifying frequently accessed datasets versus those that are rarely needed enables better cost control. Implementing data archiving strategies can also contribute to cost efficiency, allowing businesses to retain necessary data while minimizing retrieval expenses.
Data Governance and Security Expenses
Data governance and security are critical for any organization dealing with sensitive or regulated information. Implementing security measures, such as data encryption, user authentication, and compliance with regulations, will influence costs. These expenses can vary widely based on the chosen solutions and how they scale with data growth.
Moreover, investing in sophisticated data governance tools can minimize risks and adhere to compliance requirements, which can save costs in the long run by avoiding potential fines or breaches. Organizations should allocate sufficient budget for securing their data lakes effectively.
Integration and Maintenance Costs
Integration with existing systems is pivotal for operational efficiency but can lead to additional costs. The complexity of integrating different data sources into a data lake may necessitate hiring specialized personnel or investing in advanced tools, which can influence overall expenses. Maintenance of the data lake infrastructure also incurs ongoing costs, including updates and scaling needs, that organizations must be prepared for.
Regular monitoring and maintenance are vital to ensure optimal performance but may require dedicated resources. Organizations should factor these into their cost assessments to avoid budget shortfalls over time.
Understanding these factors enables businesses to make better decisions. Each aspect has implications on both immediate and future financial commitments, and keen awareness of these elements is necessary for managing effective data lake investments.
Comparative Analysis of Data Lake Pricing from Leading Providers
Understanding the landscape of data lake pricing is essential for organizations looking to make informed investments. A comparative analysis of pricing across leading providers assists in evaluating the benefits and drawbacks of each service. Different providers have unique pricing structures, features, and levels of support. Thus, knowing what is on the market can guide decision-makers toward a solution that best aligns with their business objectives.
Pricing not only influences budgetary considerations, but it can also impact performance and scalability. Evaluating the pricing models allows organizations to discern how costs may evolve over time, especially in light of growing data needs. In this section, we will examine the pricing strategies for major players in the data lake field: Amazon Web Services, Microsoft Azure, Google Cloud Platform, and IBM Cloud.
Amazon Web Services (AWS)
Amazon Web Services offers a range of data lake solutions, most notably through Amazon S3, which is one of the core components of its pricing structure. The pricing model includes consumption-based fees, where organizations are billed based on the amount of data stored and transferred.
Key Considerations:
- Storage Costs: AWS charges per gigabyte (GB) stored monthly. Costs can vary based on the storage class selected, with lower costs available for infrequently accessed data.
- Data Transfer Fees: Moving data in and out of AWS can incur additional fees, especially for outbound data transfer.
- Request Costs: There are costs associated with the number of requests made to S3, which can add up depending on usage patterns.
- Scalability: AWS provides flexible pricing and scalability options, which can suit both small and large data requirements effectively.
Microsoft Azure
Microsoft Azure’s data lake solution is anchored in Azure Data Lake Storage, designed specifically for analytics purposes. Their pricing also follows a pay-as-you-go model. Azure’s focus on enterprise integration can deliver added value for organizations using Microsoft products.
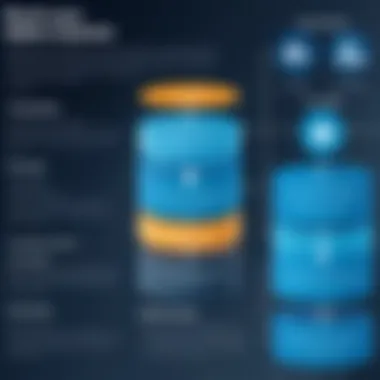
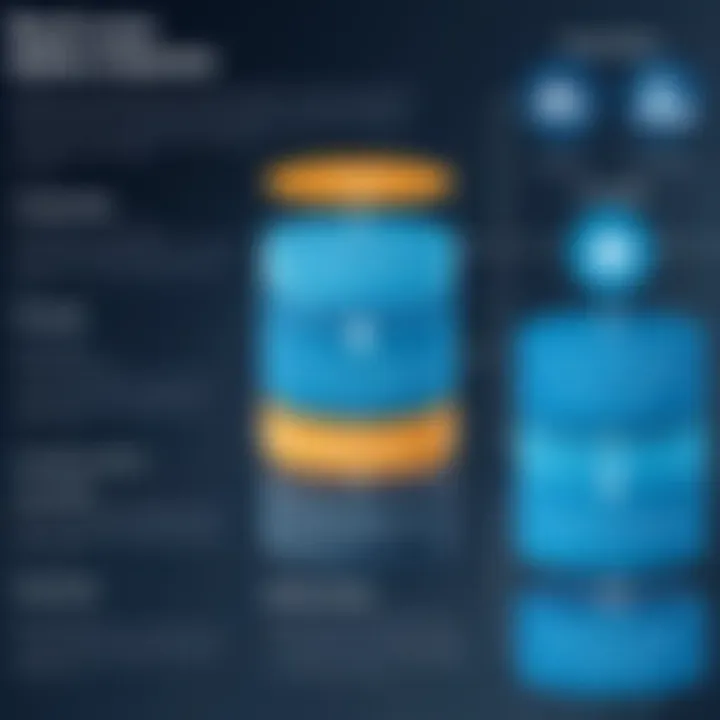
Key Considerations:
- Storage Costs: Like AWS, Azure has tiered pricing based on performance and access frequency, with lower costs for cool or archive storage.
- Data Transfer: There are charges for data egress, which can impact costs for businesses that need to regularly move large data sets.
- Analytics Integration: Azure offers bundled services, which may simplify user experience but can complicate understanding total costs if not monitored.
- Enterprise Solutions: Their integration with other Microsoft services often appeals to enterprises already invested in their ecosystem.
Google Cloud Platform
Google Cloud Platform uses Google Cloud Storage to provide its data lake services. Their pricing model also adopts a consumption-based approach but is distinguished by its overall simplicity.
Key Considerations:
- Transparent Pricing: Google Cloud provides a straightforward pricing model, which many users find easy to navigate compared to competitors.
- Storage Classes: Various storage classes are available to cater to different access needs, optimizing costs based on data retrieval frequency.
- Networking Costs: Google charges based on network egress, similar to other providers, adding another layer to cost considerations.
- Unified Data Services: Google’s ability to integrate with its data analytics services offers advantages to organizations focused on comprehensive data solutions.
IBM Cloud
IBM Cloud's offering includes IBM Cloud Object Storage as a solution for data lakes. Known for a strong emphasis on security, IBM appeals to industries with high compliance requirements.
Key Considerations:
- Unique Pricing Arrangement: IBM has a simplified pricing structure designed to support both small and large datasets.
- Security Features: Enhanced security measures have associated costs that organizations must take into account.
- Flexibility: IBM offers various plans that can adapt to different data needs and budgets, but users should carefully analyze the full cost of ownership.
- Support & Services: IBM’s customer support may be included in the pricing structure, providing distinct advantages for companies requiring additional assistance.
Analyzing the Total Cost of Ownership (TCO) for Data Lakes
Understanding the Total Cost of Ownership (TCO) for data lakes is crucial for organizations considering an investment in such infrastructure. TCO encompasses more than just the initial expenses of setting up a data lake. It includes various recurring costs associated with ongoing operations, maintenance, and future scalability. An accurate TCO analysis can empower decision-makers to estimate budget requirements and foresee long-term financial commitments accurately.
Key Elements in TCO Analysis
Analyzing TCO for data lakes involves several specific elements:
- Initial Set-Up Costs: This includes expenses related to the procurement of hardware, software licenses, and any specialized services required for implementation.
- Operational Costs: Ongoing operational expenses such as personnel, data storage, and maintenance fees must also be factored in.
- Future Scalability: Evaluating potential growth and the costs associated with scaling the data lake system as business needs evolve is essential.
By performing a comprehensive TCO analysis, organizations can prevent unforeseen costs and ensure that their investments align with long-term objectives.
Calculating Initial Set-Up Costs
The first step in determining TCO is to calculate the initial set-up costs associated with establishing a data lake. This can include several components such as:
- Infrastructure Costs: Depending on whether the data lake is deployed on-premises or in the cloud, expenses will vary. On-premises solutions often require substantial hardware investments.
- Software Licenses: Many data lake solutions have associated licensing fees. These fees can vary based on the scale of deployment and the tools chosen.
- Professional Services: External consulting or implementation services can add to initial expenses. Engaging experts to configure systems correctly may save costs in the long run.
Organizations must thoroughly research and budget for these aspects to avoid budgeting failures at the outset.
Evaluating Long-Term Operational Costs
Once initial set-up costs are understood, evaluating long-term operational costs is next. This includes:
- Storage Fees: Continuous data growth leads to increased storage costs, particularly with high volumes of unstructured data typical in data lakes.
- Data Transfer Fees: Transferring data in and out can incur significant costs, especially if the lake operates in a cloud-based environment.
- Personnel Expenses: Skilled personnel are vital for maintaining data lakes. Salaries for data engineers, analysts, and security experts should be part of recurring operational cost assessments.
It’s important to deepen operational cost understanding to create accurate forecasts that ensure financial viability over time.
Assessing Scalability and Future Investments
When analyzing TCO, assessing scalability is a necessary step. Companies must consider:
- Future Storage Needs: As data volumes increase, storage scalability is vital. Organizations should plan for future growth in data ingestions.
- Technological Upgrades: The data landscape evolves rapidly; considering potential expenses for technology upgrades can ensure effective adaptations.
- Expanding Use Cases: As business needs grow, new use cases for the data lake may emerge, and additional investments in features may arise.
Planning for future investments allows better alignment between current capabilities and expected growth, safeguarding against costly surprises later on.
Powerful insight into TCO simplifies decision-making when implementing data lakes, ensuring investments yield positive returns in the long term.
Cost Optimization Strategies for Data Lakes
Cost optimization is crucial when dealing with data lakes. As organizations increasingly turn to data lakes for storage and analysis, managing costs effectively becomes a key consideration. By adopting various optimization strategies, organizations can reduce their expenditures significantly, ensuring that their investment in data management pays off in the long run. The benefits include improved budget adherence, enhanced data accessibility, and ultimately, better decision-making capabilities.
Choosing the Right Storage Solutions
Selecting the appropriate storage solution is fundamental to cost management in data lakes. There are several options available, each with its own cost implications.
- On-Premises vs. Cloud Solutions: On-premises storage may have high upfront costs but can reduce ongoing expenses. In contrast, cloud solutions, like Amazon S3 or Google Cloud Storage, can provide scalable options without large initial investments. Understand which structure aligns better with your financial strategy.
- Storage Tiering: Implementing tiered storage can help optimize costs. Frequently accessed data can be stored in faster, more expensive storage, while less critical information can reside in lower-cost options. This method ensures that businesses only pay for the performance they require at any given time.
Implementing Data Retention Policies
Data retention policies are essential to control costs. Without clear guidelines, organizations may end up storing redundant or outdated data, increasing costs without any value return.
- Establishing Clear Guidelines: Determine what data is necessary for compliance, analytics, and operational needs, and set up timelines for data deletion.
- Regular Audits: Conduct regular data audits to identify and remove unnecessary data. This practice not only lowers storage costs but also enhances performance and quickens data retrieval.
- Archiving Strategies: Implement archiving policies that move older, less-used data to cheaper storage options. This frees up primary resources for current projects while keeping important information accessible when required.
Leveraging Automation and Monitoring Tools
Finally, the adoption of automation and monitoring tools plays a significant role in cost optimization.
- Automated Data Cleanup: Automated scripts can delete or archive data that no longer meets retention criteria. This reduces manual effort and minimizes human error.
- Monitoring Usage Patterns: Tools that analyze data access trends can help in deciding resource allocation more effectively, ensuring that the organization saves on unnecessary costs.
- Integration of AI: Using AI to provide insights into data management can lead to more informed decisions regarding resource usage.
"Investment in optimization tools and strategies pays off when it leads to smarter data management and reduced operational costs."
Through these strategies, organizations can not only reduce costs but also enhance the overall effectiveness of their data lakes. Understanding the nuances and implementing these techniques will help data managers ensure sustainable data practices while meeting business objectives.
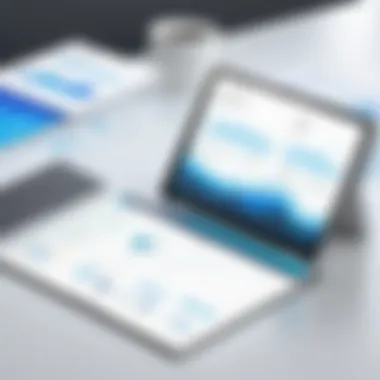
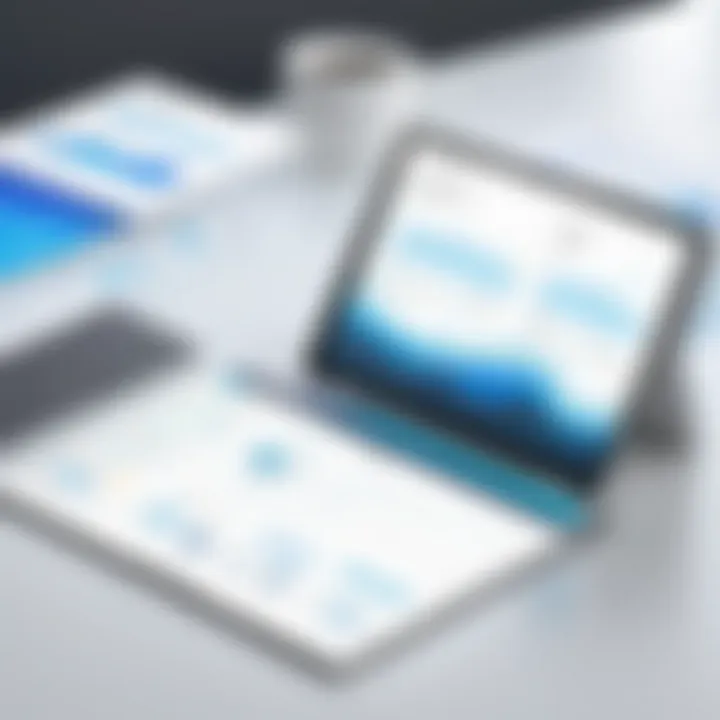
Future Trends in Data Lake Pricing
The landscape of data lake pricing continues to evolve. As organizations increasingly rely on data lakes to manage large volumes of data, understanding future trends is crucial. These trends affect not only costs but also how businesses plan their data strategies. Changes in regulations, the rise of hybrid solutions, and advancements in architecture play significant roles in shaping data lake pricing practices.
Impact of Changing Data Regulations
New regulations related to data privacy and usage are being established worldwide. Laws like the General Data Protection Regulation (GDPR) in Europe and the California Consumer Privacy Act (CCPA) in the United States mandate strict rules for data handling. Businesses must invest in compliance measures to avoid heavy penalties. This situation results in increased operational costs for data lakes.
Considerations for companies include:
- Compliance costs: Allocating funds for legal counsel and audits.
- Data management practices: Modifying how data is collected, stored, and processed.
- Privacy technologies: Implementing tools to ensure secure data handling.
These expenses impact the overall budget for data lake operations, making awareness of regulations essential for accurate cost forecasting.
Emergence of Hybrid and Multi-Cloud Solutions
The shift towards hybrid and multi-cloud environments suggests another evolution in pricing. A hybrid cloud combines public and private resources, offering greater flexibility for data management. Organizations are looking for ways to optimize their data handling without being locked into a single vendor.
Some benefits include:
- Cost efficiency: Selecting services based on specific needs can reduce expenses.
- Business agility: Quick adaptation to changing technologies or market demands.
- Data locality: Storing data closer to its source to improve performance and compliance.
However, managing multiple environments can complicate pricing structures. Companies must consider:
- Integration costs: Ensuring seamless operation across platforms.
- Data transfer fees: Costs associated with moving data between providers.
Advancements in Data Architecture
Innovations in data architecture are set to impact pricing strategies. Techniques like data mesh and lakehouse models encourage a more decentralized approach to data management. This shift can lead to reduced costs associated with traditional data lakes.
Key advancements now influencing pricing are:
- Increased efficiency: Improved architectures can lower storage and processing costs.
- Enhanced performance: Faster data access reduces resource usage.
- Scalable solutions: Organizations can grow their capacity without incurring substantial costs.
These advancements necessitate continual evaluation of pricing frameworks to optimize spending while maximizing data utility.
Understanding these future trends is essential for informed decision-making regarding data lake investments. Organizations that adapt proactively can avoid unnecessary costs and leverage their data more effectively.
Real-World Case Studies
Case studies serve as a pragmatic lens through which stakeholders can understand the real-world applications and implications of data lake investments. They reflect actual outcomes, bringing theory into context. Decision-makers often face challenges around estimating costs and benefits of implementing a data lake. Therefore, examining how other organizations navigate these waters provides valuable insights.
In this section, we will explore two distinct case studies. One will focus on a small business implementation, while the other will delve into considerations faced by large enterprises. These narratives are vital for grasping how various factors affect costs and ultimately the value derived from a data lake solution.
Cost Analysis of a Small Business Implementation
Small businesses often face unique challenges when it comes to adopting data lake technology. Budget constraints and limited resources can complicate decision-making. The implementation of a data lake in a small business context often involves several factors:
- Initial Setup Costs: These encompass hardware, software, and the necessary cloud services. Small businesses may choose to adopt a cloud-based solution, thus minimizing up-front infrastructure costs.
- Ongoing Storage and Data Transfer Costs: The choice of storage solutions can significantly impact long-term expenditures. Small businesses might deal with fluctuating data volumes, affecting the pricing based on usage.
- Maintenance and Integration Expenses: Regular maintenance is critical for ensuring data integrity and performance. Small businesses might opt for pre-built solutions that require less integration effort, although this may limit customization.
In a case examined, a regional online retail store implemented a data lake. The decision led to improved data insights on customer behavior, but the small team faced challenges in fully utilizing the capabilities of the data lake without adequate technical expertise. Balancing these factors is crucial for small businesses looking to maximize their data investments.
Large Enterprise Cost Considerations
When it comes to large enterprises, the landscape of data lake pricing shifts. These organizations often operate at scale, which can introduce both advantages and complexities in cost management.
- Scalability Costs: Large enterprises generally deal with massive amounts of data. This volume influences storage cost, data transfer fees, and necessary infrastructure investments.
- Customization Needs: Larger organizations often require tailored solutions that align with their operational needs. This can lead to higher initial development costs but may yield better long-term results.
- Risk Management Expenses: Data governance and security are paramount. Enterprises must consider compliance in data management, which can add additional layers of cost.
One notable example is a multinational financial institution that adopted a data lake for integrating diverse data sources. Although they faced higher setup and operational costs relative to their smaller counterparts, the enterprise benefited from enhanced risk analysis and regulatory compliance.
Thus, large enterprises must weigh the initial costs against the long-term benefits, ensuring that the data lake aligns with strategic goals. The outcome from such implementations often guides future decisions regarding data management within similar sectors.
Closure: Making Informed Decisions on Data Lake Investments
Making informed decisions regarding data lake investments is critical in today’s data-centric landscape. Businesses increasingly rely on data lakes to harness vast amounts of data for analytics, machine learning, and artificial intelligence. However, understanding the cost implications of these investments is equally essential.
Importance of Understanding Pricing
Data lake pricing is not simply about finding the lowest cost provider. It involves a multifaceted analysis of various pricing models and their impact on overall budget. Knowing the difference between consumption-based, subscription-based, and tiered pricing can influence how a business plans its data management strategy. Each model has its merits and drawbacks, affecting long-term operational costs and resource allocation.
Consideration of Hidden Costs
Often, organizations overlook hidden costs associated with data lakes. These can include data transfer fees, access and retrieval charges, and expenses tied to data governance and security. A thorough analysis should consider all potential fees. For instance, poor data governance can lead to unforeseen fines or loss of data integrity, which can be more costly than the initial set-up.
Strategic Evaluation of Needs
Before making any investment, businesses should conduct a thorough needs assessment. This includes evaluating the volume of data, frequency of access, and compliance requirements. A data lake must align with the strategic goals of the organization.
Final Thoughts on Budgeting
A sound budgeting process that incorporates initial set-up costs, long-term operational expenses, and future scalability requires attention to detail. Tracking expenditure and optimizing resource usage can lead to significant savings.
To conclude, the decision to invest in a data lake should not be rushed. It involves careful consideration of pricing models, potential hidden costs, and alignment with organizational objectives. By making informed choices, organizations can maximize their investment in data lakes while minimizing financial risks.
"An informed decision is a well-calculated risk; it is about maximizing benefit while controlling costs."
By understanding data lake pricing deeply, businesses can navigate complexities and make decisions that not only support current needs but also facilitate future growth.