Understanding Data Collection and Analysis Companies
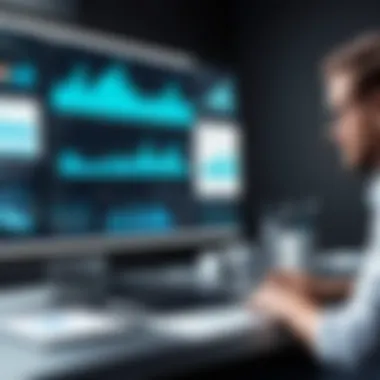
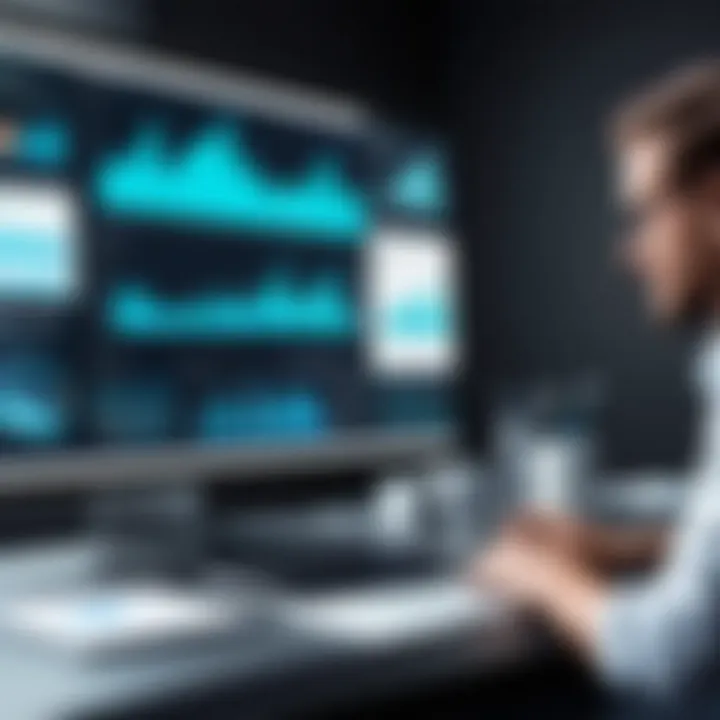
Intro
Data collection and analysis companies play a crucial role in today's business environment. They gather and interpret data to provide insights that help organizations make informed decisions. Understanding this industry is essential, especially for small and medium-sized business owners looking to leverage data effectively. This section will outline the core features of these companies and highlight the tools and methodologies they employ.
Overview of Key Features
The proficiency of data collection and analysis companies lies in their software capabilities. These capabilities directly influence the quality of insights derived from data. Here are some key aspects to consider:
- Data Integration: The ability to combine data from different sources is vital. Companies use advanced tools to integrate data from social media, sales, and customer interactions.
- Data Visualization Tools: Good visual representation of data is fundamental. Sophisticated visualization helps in identifying trends and patterns easily.
- Analytics Engine: An analytics engine processes the data. It employs algorithms and statistical methods to deliver meaningful insights.
- Real-Time Processing: Businesses demand real-time data analysis. Companies providing these services often implement cloud computing technologies to accommodate this necessity.
Unique features can set certain companies apart from their competitors. For instance, customizable dashboards allow users to tailor their data views according to their specific needs. Additionally, some companies focus on predictive analytics, offering forecasts that aid decision-making processes.
User Experience
The user experience is a significant factor in how effective these tools are for businesses. A user-friendly interface encourages wider adoption within an organization.
- Interface and Usability: Data analysis software should be intuitive and accessible. Complicated tools can alienate users who lack technical backgrounds. Clear navigation and ease of use are essential features.
- Support and Community Resources: Reliable customer support can enhance overall experience. Many companies offer extensive resources through forums, online tutorials, and customer service teams. Engaging communities can serve as invaluable resources for best practices and troubleshooting.
Fact: According to a recent study, organizations utilizing analytics are five times more likely to make faster, better decisions.
In summary, understanding the key features and user experience of data collection and analysis companies will empower business professionals to make educated choices about the services they utilize. This foundational knowledge is the first step towards leveraging data effectively in their operations.
Prologue to Data Collection and Analysis
Data collection and analysis form the backbone of many business strategies today. As companies evolve, understanding these processes is essential. Data is no longer just numbers; it is an invaluable resource that helps drive decisions.
Defining Data Collection
Data collection is the systematic approach of gathering information to generate meaningful insights. This process encompasses various methods, such as surveys, interviews, and observational techniques. When executed well, data collection yields information that can influence product development, marketing strategies, and operational workflows. The clarity of purpose in data collection ensures that the data gathered is relevant and serves a defined business objective.
Different sectors utilize data collection techniques effectively based on their unique needs. For instance, in healthcare, patient feedback is gathered through tailored surveys to improve services. In retail, consumer behavior is analyzed through loyalty programs. The right data collection method can provide deep insights that are aligned with specific goals, enabling firms to strategize better.
Defining Data Analysis
Data analysis refers to the processes of inspecting, cleansing, and modeling data to discover useful information. It goes hand in hand with data collection and serves to transform raw data into valuable insights. Techniques can vary widely, from simple statistical analysis to advanced machine learning algorithms.
Analyzing data allows businesses to identify trends, correlations, and anomalies. For example, through cohort analysis, a company can understand the behavior of a group of customers over time, adjusting offerings according to their preferences. Likewise, analysis can help determine the effectiveness of marketing campaigns by quantifying customer engagement and conversion rates.
The efficiency of data analysis hinges on selecting the appropriate tools and methodologies. Software solutions such as Tableau or Microsoft Power BI have gained popularity for their visualization capabilities, making complex data more digestible. Furthermore, predictive analysis can forecast future trends based on historical data, guiding strategic planning.
The convergence of data collection and analysis is crucial for effective decision-making. Companies that master these domains position themselves for sustained success.
Importance of Data in Decision Making
The role of data in decision making cannot be overstated. In an age where information dictates competitive advantages, understanding the nuances of data collection and analysis becomes crucial for businesses. Accurate and relevant data informs strategies, optimizes resources, and improves overall efficiency.
Data-Driven Decision Making
Data-driven decision making refers to the process of making systematic decisions based on data analysis rather than intuition. This approach enables organizations to forecast trends, measure performance, and assess risks effectively. Businesses that leverage data can respond to market shifts, customer preferences, and operational efficiencies much faster than their competitors.
Key benefits of data-driven decision making include:
- Improved Accuracy: By basing decisions on concrete data, organizations minimize the risk of errors associated with guesswork.
- Enhanced Insights: Data analysis reveals patterns and trends that might not be immediately apparent, leading to more informed choices.
- Increased Accountability: When decisions stem from data, it is easier to hold teams accountable for outcomes, fostering a culture of measurement and improvement.
Case Studies: Successful Applications
To illustrate the power of data in decision making, we can look at examples from prominent organizations.
Case Study One
A notable application of data-driven decision making can be seen in Netflix. The streaming giant relies heavily on audience data to drive its content recommendations and production decisions. By analyzing viewing habits and user ratings, Netflix not only optimizes user experience but also strategically invests in original content.
What makes Netflix’s approach beneficial is its commitment to personalization. The algorithms used categorize viewers into segments, allowing for tailored recommendations. This unique feature contributes to increased engagement and customer satisfaction. However, the dependence on algorithms can lead to potential bias, as the data reflects existing trends rather than encouraging diverse content creation.
Case Study Two
Another example is Amazon, which utilizes data extensively to refine its inventory management and sales strategy. By analyzing consumer purchasing patterns and preferences, Amazon optimizes stock levels and enhances supply chain efficiency. This application leads to improved customer satisfaction through timely deliveries.
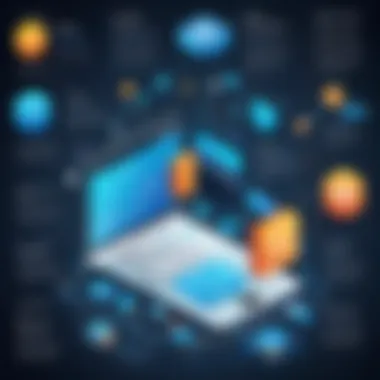
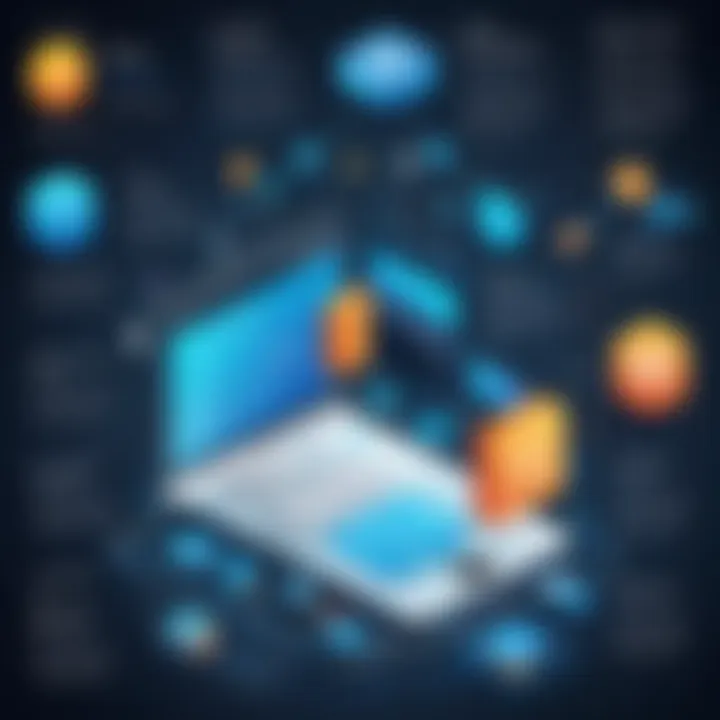
The key characteristic of Amazon’s data approach is its ability to predict consumer behavior accurately. This predictive capability enables the company to offer personalized shopping experiences. While this feature is advantageous for driving sales, it can also lead to concerns about privacy, as extensive data collection raises questions about consumer consent and data ownership.
"Data-driven insights empower organizations to navigate complexities with clarity and confidence."
In summary, the importance of data in decision making is amplified by real-world examples. By understanding and utilizing data, companies can enhance their strategic initiatives and maintain relevance in a competitive environment.
Types of Data Collection Techniques
Data collection techniques are crucial as they form the foundation of quality data. The choice of method can significantly influence the accuracy and reliability of the information gathered. By understanding various techniques, organizations can select the ones that best fit their specific needs. This section focuses on the importance of several types of data collection methods, highlighting the benefits and considerations involved in each.
Surveys and Questionnaires
Surveys and questionnaires remain one of the most prevalent data collection methods. These tools allow organizations to gather data directly from respondents. They are particularly useful for collecting quantitative data that can be easily analyzed. The design of these instruments can vary greatly, affecting the quality of the data collected.
Key benefits include:
- Wide Reach: Surveys can reach a large audience quickly, especially through online platforms.
- Cost-Effectiveness: Conducting surveys can be relatively inexpensive compared to other methods.
- Data Standardization: Responses can be easily quantified, allowing for straightforward data analysis.
However, there are considerations to keep in mind. Poorly designed surveys may lead to biased results. It’s essential to craft clear and impartial questions to mitigate this risk. Furthermore, distribution methods can impact data quality—ensuring participants are representative of the target population is crucial.
Observational Methods
Observational methods involve watching subjects in their natural environment without interaction. This technique is invaluable for gathering qualitative data and can provide insights that surveys may overlook. These methods are often used in social sciences, behavioral studies, and market research.
Advantages of observational methods include:
- Real-Time Data: Observers can capture data as it happens, reflecting authentic behavior.
- Contextual Understanding: Contextual factors are integrated into data collection, leading to a richer understanding of subjects.
Nonetheless, observational techniques have challenges. The observer’s bias can influence interpretations. Moreover, resource requirements can be significant, demanding time and trained personnel.
Digital Data Collection
Digital data collection has gained prominence due to technological advancements. This method utilizes tools and platforms—such as web forms or mobile applications—to collect data efficiently. The growth of big data analytics has made digital collection even more vital in understanding user behavior and preferences.
Benefits include:
- Efficiency: Digital tools streamline the data gathering process, reducing time and effort.
- Accessibility: Data can be collected from various sources, including social media and online platforms.
- Automation: Many tools allow for automatic data compilation and analysis, enhancing accuracy.
One must also consider the challenges. Data privacy concerns are significant, as sensitive information is often collected. Compliance with regulations, such as the General Data Protection Regulation (GDPR), is imperative. The technology used must ensure secure data handling to maintain user trust.
In summary, the selection of data collection techniques must align with specific goals and context, ensuring both quality and ethical standards.
Major Players in Data Collection and Analysis
The data collection and analysis industry is vast and intricate, housing numerous organizations that specialize in various methodologies and technologies. Understanding who the major players are in this sector can provide valuable insights for small to medium-sized businesses aiming to harness the power of data for their strategies. These companies not only drive innovation but also influence trends across multiple sectors, including healthcare, finance, and marketing. They are the backbone of most data-driven decisions. The importance of recognizing leading companies and niche providers lies in their ability to deliver specific capabilities and solutions that can transform businesses.
Leading Companies
Leading companies in data collection and analysis typically stand out due to their size, reach, and comprehensive solutions. Notable organizations like Nielsen, Ipsos, and Gartner have established reputations for effective data collection methods, including surveys, market assessments, and consumer insights. These firms possess extensive resources and technologies that enable them to process and analyze data efficiently.
The benefits of working with these leading companies include:
- Access to Expertise: Their experience allows them to handle complex data needs and challenges.
- Comprehensive Services: They provide a broad range of services from data collection to analysis, ensuring seamless integration of processes.
- Scale and Coverage: Often, these companies can operate globally, collecting data across diverse markets, providing businesses with relevant insights tailored to different geographical areas.
- Established Methodologies: Their proven methodologies and frameworks offer businesses reliable strategies for data collection and analysis.
Working with leading companies means getting the advantage of seasoned professionals who understand the nuances of data collection and the implications of their analysis.
Niche Providers
Niche providers fill critical gaps in the data collection and analysis landscape. These companies specialize in specific areas or sectors, offering tailored solutions that may not be available from larger leading firms. Providers such as Qualtrics, SurveyMonkey, and Typeform exemplify the diverse methodologies present within this niche.
The main advantages of niche providers include:
- Specialization: They often possess deep knowledge in their area of focus, enabling a more tailored approach to data needs.
- Innovative Solutions: Niche companies frequently push the envelope with innovative tools that address specific challenges more effectively.
- Flexibility and Customization: They are typically more agile, allowing for customization to meet the unique needs of their clients.
- Cost-Effectiveness: Smaller scale operations can translate into more competitive pricing compared to larger firms.
Technological Advancements in Data Analysis
Technological advancements play a crucial role in the landscape of data collection and analysis. These advancements enhance the efficiency, accuracy, and scalability of data operations. As businesses increasingly rely on data-driven strategies, understanding these technologies becomes essential for decision-makers. New tools continuously emerge, shaping how companies approach data collection and analysis, ultimately influencing their operational strategies.
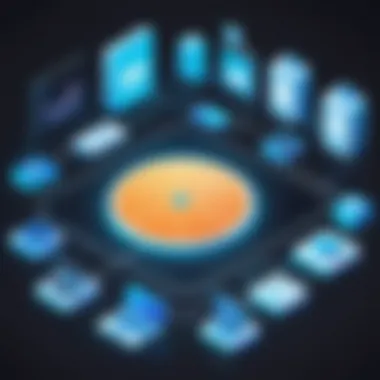
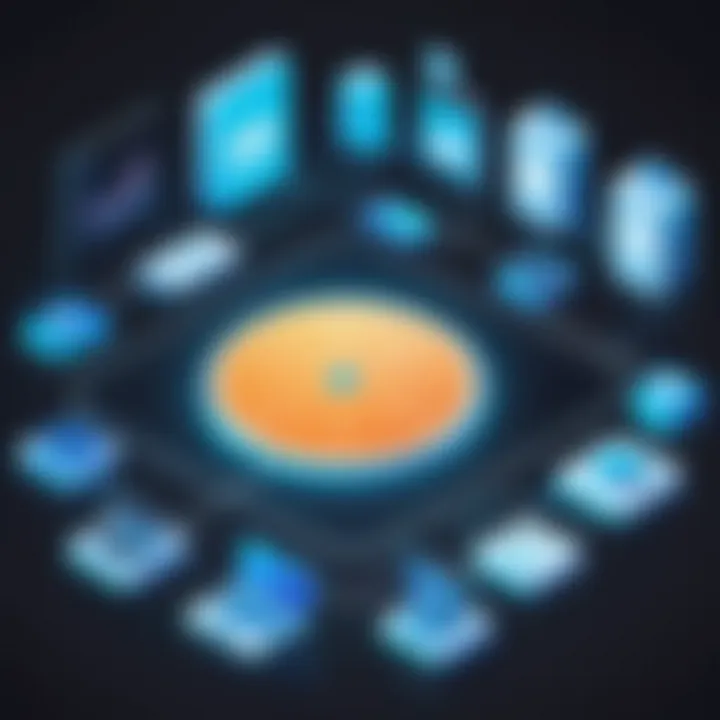
Artificial Intelligence and Machine Learning
Artificial Intelligence (AI) and Machine Learning (ML) are transformative technologies in data analysis. These technologies allow for the automation of complex processes that would typically require significant human effort. For instance, AI algorithms can optimize data sorting and filtering, making it quicker to identify patterns and trends. This benefit empowers organizations to make decisions based on real-time insights rather than historical data alone.
Moreover, ML models improve over time as they process more data, leading to increased accuracy in predictions. This characteristic allows businesses to respond dynamically to changes in data patterns. Companies utilizing AI and ML can gain competitive advantages by identifying market trends ahead of their rivals. In sectors like finance and healthcare, where data-driven decisions are vital, the implementation of AI and ML can lead to substantial improvements in outcomes.
Big Data Technologies
Big data technologies are essential in handling vast datasets that traditional data management tools cannot efficiently process. The emergence of tools like Apache Hadoop and Apache Spark has revolutionized how companies store and analyze large volumes of data. These tools allow for distributed computing, enabling organizations to process data across multiple servers simultaneously, drastically reducing analysis time.
With big data technologies, businesses gain the ability to handle diverse data types, including structured, semi-structured, and unstructured data. This versatility means organizations can analyze everything from customer transaction records to social media interactions. As a result, businesses gain a holistic view of their operations, leading to better strategic decisions.
"Employing big data technologies allows companies to leverage analytics at an unprecedented scale, unlocking new opportunities for innovation and insight."
Organizations embracing big data technologies often report enhanced customer experiences and improved operational efficiency. By integrating these technologies into their data strategies, businesses can not only stay relevant in their industries but also lead the charge towards innovation. As technology continues to advance, the integration of AI, ML, and big data will undoubtedly shape the future of data collection and analysis.
Ethical Considerations in Data Collection
Ethical considerations are fundamental to the practice of data collection and analysis. In a world where data is abundant and ever-growing, the implications of collecting and using data without regard for ethics can be severe. Organizations are tasked with ensuring that individual rights are respected and that their data practices do not compromise the privacy of consumers. When companies adhere to ethical guidelines, they bolster trust among their clients and consumers, essential for sustaining success in the competitive landscape of data services.
A crucial element of ethical considerations is safeguarding privacy and confidentiality. Consumers are increasingly aware of their rights regarding personal data, compelling companies to implement robust measures to protect sensitive information. This goes beyond legal compliance; it is about building a culture of respect and responsibility toward individuals whose data might be collected. Managing data ethically can result in improved relationships with consumers, thereby fostering loyalty and enhancing brand reputation.
Additionally, adherence to regulations is vital in this sphere. Regulatory frameworks such as the General Data Protection Regulation (GDPR) and the California Consumer Privacy Act (CCPA) have established essential guidelines for how data can be collected and processed. These regulations not only impose restrictions but also set a benchmark for ethical conduct, promoting transparency and accountability in data practices.
Overall, ethical considerations not only help companies avoid potential lawsuits but also serve to create a more equitable data ecosystem. This aligns organizational practices with the expectations of a more informed and privacy-conscious public.
Privacy and Confidentiality
Privacy and confidentiality are at the forefront of ethical considerations in data collection. Organizations must recognize that data security is not merely a technical requirement; it involves a moral obligation to individuals to whom the data pertains. When companies collect data, they often gather personal information that can expose individuals to various risks, including identity theft and unauthorized access to personal details.
To mitigate these risks, organizations need to implement stringent measures to protect privacy. This includes data encryption, access controls, and regular audits of data management processes. Furthermore, organizations should be transparent about how data is collected, stored, and used. By offering clear information regarding their practices, companies can foster a sense of security among their users, emphasizing their commitment to ethical standards.
Compliance with Regulations
GDPR
The General Data Protection Regulation, or GDPR, is one of the most significant legislative efforts aimed at protecting personal data. It was established to enhance individual rights and provide citizens with greater control over their personal information. A key characteristic of GDPR is its emphasis on informed consent. Organizations must clearly explain what data they collect and the purposes for which it will be used, ensuring that individuals can make informed decisions about their data.
The GDPR is a beneficial choice for this article because it redefines standards for data protection globally. It sets expectations not only for companies operating in the European Union but also for any organization that interacts with EU citizens. This increased scrutiny ensures that companies maintain high ethical standards in their data practices.
One unique feature of GDPR is the requirement for organizations to appoint a Data Protection Officer (DPO). The DPO’s role is essential for overseeing compliance and ensuring that data handling practices align with regulations. This adds an extra layer of accountability, fostering public trust and ensuring ethical conduct at all levels of the organization.
CCPA
The California Consumer Privacy Act, or CCPA, complements GDPR by providing California residents with specific rights regarding their personal data. It allows individuals to know what personal data is being collected and gives them the right to request deletion of their data. A key characteristic of CCPA is its applicability to for-profit businesses that collect consumer data, making it a critical regulation for many organizations operating in California.
CCPA is also significant for this article because it represents a shift toward consumer empowerment in data privacy. It emphasizes the notion that individuals should have control over their data and how it is used. Moreover, it encourages companies to adopt transparent practices for data management, thus fostering ethical behavior in data collection.
One unique feature of the CCPA is its stipulation of monetary penalties for non-compliance. This makes understanding and adhering to CCPA a priority for companies that handle consumer data, as violations can be costly.
Challenges Faced by Data Collection Companies
Data collection companies play a critical role in how businesses extract insights from data. However, they face significant challenges that can impact their effectiveness. This section explores these challenges to provide a deeper understanding of their implications on data quality and overall business decisions.
Data Quality and Integrity
Data quality is paramount in the realm of data collection. Poor quality data can lead to misleading analyses, affecting decision-making processes substantially. Companies often struggle with ensuring accuracy in the data they collect due to several factors:
- Inconsistent Data Sources: Data is often gathered from various platforms, each with its own standards. This can lead to discrepancies, making it hard to maintain a single source of truth.
- Human Error: Despite technological advancements, human error is still a significant issue. Mistakes in data entry or processing can compromise data integrity.
- Validity of Data: The relevance and accuracy of collected data must also be ensured. Using outdated or irrelevant datasets can skew results. Therefore, companies must regularly review and update their data collection criteria to maintain validity.
In addition to these issues, data integrity is crucial. This refers to the accuracy and consistency of data throughout its lifecycle. Compromising integrity can lead to substantial financial losses and damaged reputations. Therefore, companies must develop robust quality control measures and strict validation processes to assure stakeholders of their data's reliability.
Technological Limitations
The technological landscape is constantly evolving, yet data collection companies often find themselves lagging behind in implementing the latest advancements. Several limitations arise as a result:
- Infrastructure Constraints: Many companies do not have the necessary technological infrastructure to process large volumes of data effectively. Insufficient hardware and software can lead to bottlenecks or failures in data processing.
- Integration Issues: Companies often face challenges integrating new data systems with their existing technologies. This can result in fragmentation, hampering a unified data collection approach.
- Skill Gaps: There is a growing demand for professionals skilled in advanced data analytics and machine learning. However, the current talent pool may not meet this demand, leading to insufficient expertise in handling complex data analysis tasks.
- Cost of Implementation: Investing in new technology is often costly. Many companies may hesitate to allocate significant budgets, fearing a lack of immediate ROI. Balancing cost with innovation is a common dilemma in this space.
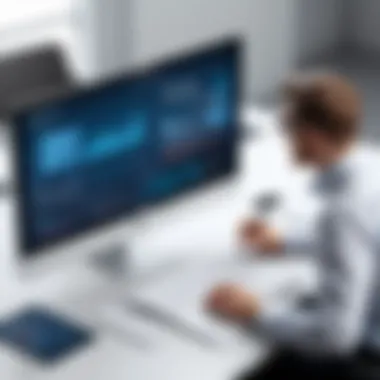
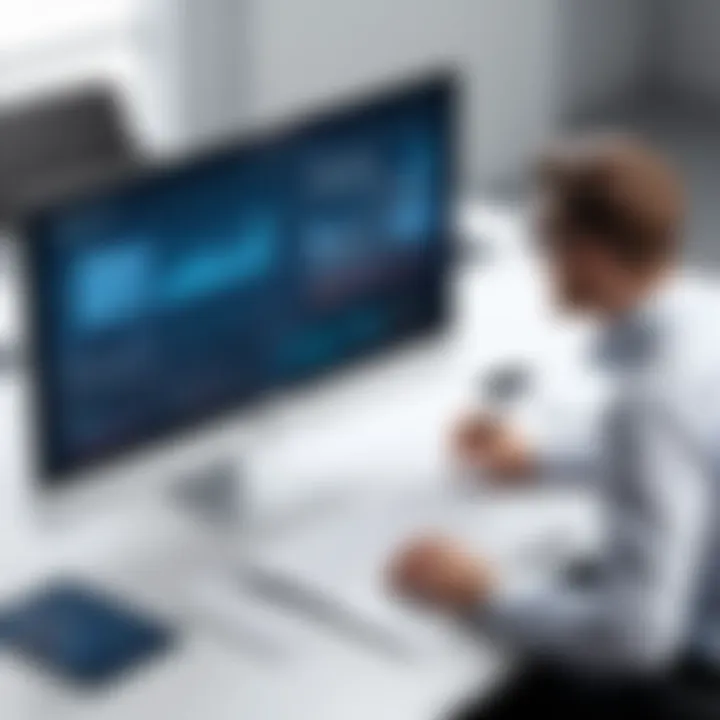
In summary, understanding these challenges is essential for any business looking to leverage data collection effectively. The focus should be on enhancing data quality and overcoming technological limitations to realize the full potential of data-driven strategies.
Collaborative Approaches and Partnerships
In the ever-evolving landscape of data collection and analysis, collaborative approaches and partnerships play a crucial role. These strategies are instrumental for data collection companies as they strive to enhance their methodologies, expand their data sources, and bolster their analytic capabilities. By forging alliances, these companies can merge their strengths with those of others, creating an ecosystem that fosters innovation, efficiency, and excellence.
Industry Collaborations
Industry collaborations involve partnerships between companies within the data collection sector or between data companies and businesses from other sectors such as marketing, healthcare, and technology. These collaborations can lead to significant advantages, including:
- Shared Resources: Companies can exchange tools and technologies to enhance their data collection processes. This sharing often results in improved data quality and comprehensive insights.
- Skill Diversification: By collaborating with firms that possess different expertise, companies can augment their knowledge base and skill set. For example, a data analytics firm partnering with a social media company can gain insights into digital user behavior.
- Market Access: Collaborations can open doors to new markets, enabling companies to access diverse consumer groups and gather rich data. This can lead to more effective strategies tailored to various demographics.
- Cost-Efficiency: Joint ventures often reduce operational costs by merging efforts in research, technology development, and marketing. This shared financial burden makes it feasible to invest in advanced technologies like machine learning.
Collaboration can also lead to improved credibility and trust among consumers. When reputable companies come together, they can establish a strong reputation, thereby attracting more clients.
Cross-Sector Relationships
Cross-sector relationships refer to partnerships between data collection and analysis companies and organizations in different fields. For instance, a health data analytics firm might collaborate with a pharmaceutical company to enhance clinical research data collection. This type of relationship can produce various benefits:
- Innovative Solutions: Cross-sector partnerships can lead to innovative solutions that neither sector could achieve alone. For example, pairing technology firms with healthcare organizations can enhance telehealth data collection methods.
- Broadening Perspectives: Collaborations outside the immediate sector can provide fresh perspectives and new methodologies. Different approaches to data can improve the overall analysis and insights derived.
- Enhanced Data Utilization: When companies from different sectors work together, they can combine datasets for more robust analysis. This larger pool of data can yield deeper insights and more accurate forecasts.
- Regulatory Compliance: Collaborations can help in navigating regulatory challenges. Industry experts can share knowledge about compliance requirements, such as GDPR and CCPA, which is vital for data-dependent organizations.
"Collaborative approaches in data collection not only drive innovation but also foster trust and efficiency in data use."
In summary, collaborative approaches and partnerships are essential for companies in the data collection and analysis sectors. By working together, they can enhance their capabilities, expand their reach, and ultimately provide more value to their clients.
The Future of Data Collection and Analysis
The upcoming trajectories in data collection and analysis reveal significant transformations, reshaping how organizations and industries leverage data. Understanding this future landscape is essential for businesses aiming to remain competitive. With rapid advancements in technology and evolving consumer expectations, knowing where data collection is headed can guide business strategies and enhance decision-making processes.
Emerging Trends
In the realm of data collection and analysis, several emerging trends signal a shift in methodologies and applications. Here are some key trends to watch for:
- Increased Use of Real-Time Data: Organizations are slowly moving from retrospective data analysis to real-time processing, ensuring quicker decisions that can adapt to immediate market conditions.
- Augmented Analytics: This utilizes machine learning to automate data preparation and insight generation. As a result, even those with limited data expertise can derive meaningful insights from data sets.
- Ethical Data Practices: More companies are recognizing the importance of ethical considerations, focusing on transparency and consent in data collection. This trend is vital for maintaining consumer trust.
- Integration of IoT Devices: Internet of Things devices are increasingly being used to collect extensive data, providing real-time insights into settings ranging from smart homes to industrial applications.
These trends indicate that the future of data collection will be more complex yet more informative, necessitating an adaptation of strategies by businesses.
Predicted Changes in Technology
As we gaze into the future of technology in data collection and analysis, several predicted changes will significantly impact how data is processed and utilized:
- Advanced Machine Learning Algorithms: Algorithms will become more sophisticated, enhancing predictive analytics capabilities that enable organizations to forecast trends more accurately.
- Greater Cloud Integration: The shift towards cloud-based data management will facilitate easier access and sharing of data across organizations, fostering collaborative efforts and innovation.
- Blockchain for Data Privacy: With increased concerns regarding data security, blockchain technology could be utilized for secure data sharing that ensures integrity and privacy.
- Natural Language Processing (NLP): As tools that can interpret human language advance, data analysis will evolve. Businesses will be able to analyze qualitative data more effectively, extracting insights from unstructured data like customer feedback and social media interactions.
These technological shifts suggest a future where data collection is not just about quantity, but also quality and relevance.
"As businesses navigate these changes, staying attuned to emerging trends and adapting to technological advancements will be crucial for sustaining a competitive edge."
By keeping abreast of these trends and predicted technological changes, organizations can position themselves to harness the full potential of data collection and analysis, fostering enhanced decision-making and business growth.
The End
The conclusion serves as a pivotal element in this article. It synthesizes the information discussed, allowing readers to grasp key points and the overall importance of data collection and analysis. By highlighting crucial insights, this section reiterates the value of understanding how these companies operate and their influence on diverse sectors.
In today's data-driven landscape, businesses must recognize that data collection and analysis are not just supplemental services. They are foundational to informed decision-making. Organizations that effectively leverage data are more likely to stay competitive and innovative. This conclusion underscores the significance of grasping the methodologies, technologies, and ethical considerations linked to data handling.
Key benefits that arise from a thorough understanding of this topic include:
- Enhanced Decision-Making: Companies can rely on accurate data for strategic planning.
- Identifying Trends: Understanding the mechanisms of data analysis helps predict market shifts.
- Improved Efficiency: Insights from data can lead to optimized operations and resource allocation.
- Ethical Awareness: Knowledge of compliance requirements promotes responsible usage of consumer data.
In summary, the final section acts as both a recap and a call to action for decision-makers and business professionals. It emphasizes not just the importance of data collection and analysis in shaping future outcomes but also the need for informed criteria when selecting service providers in this field.
Recap of Key Insights
To encapsulate the essence of this article, the following key insights emerge:
- The importance of accurate data collection and sophisticated analysis methods cannot be understated.
- Ethical considerations play a vital role in shaping the policies of data collection companies.
- Industry players range from large, established firms to niche providers, each offering unique value.
- Emerging technologies like AI are enhancing the capabilities of data analysis, providing deeper insights.
- Case studies illustrate successful applications, showcasing the transformative power of data when utilized correctly.
Each of these elements reflects the growing significance of data in today's business environment. The emphasis on ethical practices, advanced methodologies, and strategic importance prepares decision-makers to engage effectively with data collection and analysis firms.
Final Thoughts on Selection Criteria
Selecting a data collection and analysis company involves careful consideration of several factors:
- Reputation and Expertise: Researching potential providers' backgrounds can provide insight into their methodologies and reliability.
- Technology Stack: Understanding the technologies employed is crucial, especially regarding compatibility with your own systems.
- Ethical Standards: Ensuring that a company adheres to privacy regulations is non-negotiable.
- Customer Service: Responsive support can delineate how well a company partners with you during and after data collection.
- Cost vs. Value: Finally, assess whether the service offered aligns with your budget and expected outcomes.
Making an informed choice will greatly enhance your organization’s capacity to integrate data into strategic framework successfully. The journey into data-driven decision-making is ongoing, and careful selection of partners can make all the difference.